For the very first time, artificial intelligence (AI) has been used to discover a new type of antibiotic.
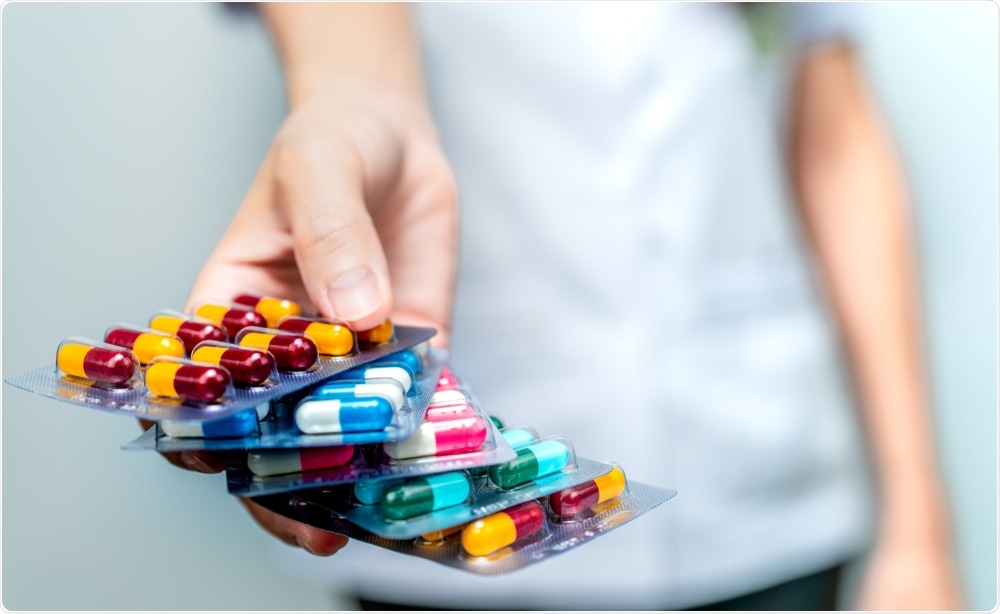
Image Credit: Fahroni/Shutterstock.com
A team from the Massachusetts Institute of Technology (MIT) published a paper last month in the journal Cell, in which they describe how they trained a deep learning model that has successfully uncovered a powerful antibiotic that can kill bacteria that is not only dangerous but also drug-resistant.
Increasing drug resistance calls for new antibiotics
Increased drug resistance has become of increasing concern in recent years. Antibiotic-resistant bacteria continue to emerge, fueling demand for new antibiotics that can fight them. Without the development of new antibiotics, their efficacy is threatened, which endangers the lives of millions of people who are saved annually by this kind of medication.
Between 2017 and 2018 the UK saw an increase of 9% of people experiencing antibiotic-resistant infections. In the US, a huge 2.8 million people suffer from this kind of infection each year, and the number continues to rise. This problem has been deemed "one of the biggest threats to global health security and development today” by the World Health Organization (WHO).
This increased demand for new antibiotics is what led MIT-based researchers to look to the advanced technological offerings of AI to address this problem.
The research
The architecture of the human brain-inspired the powerful AI algorithm that the team designed to analyze over one hundred million chemical compounds. In doing so, an artificial deep neural network, a computing system that works similar to how biological neural networks work, was trained with the capability of predicting which molecules are likely to have antibacterial activity.
The structures of over 2,500 drugs and other compounds were analyzed with the deep neural network, in search of those that had the known qualities related to toxicity to E. coli. Following this analysis, roughly 100 candidate drugs and compounds were highlighted as potentials before Halicin was identified.
Halicin, a molecule from the Drug Repurposing Hub, was picked out by the deep neural network as a potentially effective antibiotic against E. Coli. The molecule is structurally different in comparison with conventional antibiotics. It also displays bactericidal activity against a vast number of pathogens such as carbapenem-resistant Enterobacteriaceae and Mycobacterium tuberculosis.
Also, Halicin was found to be effective at treating pan-resistant Acinetobacter baumannii and Clostridioides difficile in murine models. Further to this, eight other antibacterial compounds that also have distinct structures from conventional antibiotics were also identified by the deep neural network.
The growing use of AI in pharmaceuticals
In a world-first, the MIT-based team successfully used AI to discover a new antibiotic. However, this is not the first time AI has been effectively used in pharmaceuticals. Earlier studies have seen the use of AI in similarly identifying new drug molecules.
News reported recently that for the first time, a drug molecule discovered by AI would be used in human trials. The drug is being developed to treat people with obsessive-compulsive disorder (OCD), hopefully offering them an effective new treatment option.
While the use of AI in healthcare is still in its early phases, it already shows much promise, and more breakthroughs are expected to happen in the coming years.
Recently, a paper published in the journal The Lancet claimed that AI has proved itself to be more effective at diagnosing breast cancer by studying mammograms than doctors are. In a study conducted by a team of researchers from the Imperial College London along with those from Google Health trained a computer model using X-rays taken from thousands of women to train an algorithm to spot breast cancer, which it did more accurately than six radiologists.
In the future, we will likely see more healthcare innovations grow out of developments in AI. This innovation, in particular, is expected to be used in identifying further molecules that could be effectively used as antibiotics.
Sources:
Journal reference:
- Stokes, J., Yang, K., Swanson, K., Jin, W., Cubillos-Ruiz, A., Donghia, N., MacNair, C., French, S., Carfrae, L., Bloom-Ackerman, Z., Tran, V., Chiappino-Pepe, A., Badran, A., Andrews, I., Chory, E., Church, G., Brown, E., Jaakkola, T., Barzilay, R. and Collins, J. (2020). A Deep Learning Approach to Antibiotic Discovery. Cell, 180(4), pp.688-702.e13. https://www.cell.com/cell/fulltext/S0092-8674(20)30102-1