The scale and complexity of data being thrust out of genomics has become so great that the struggle now lies with converting this data into information that is both useful and insightful.
What is genomics?
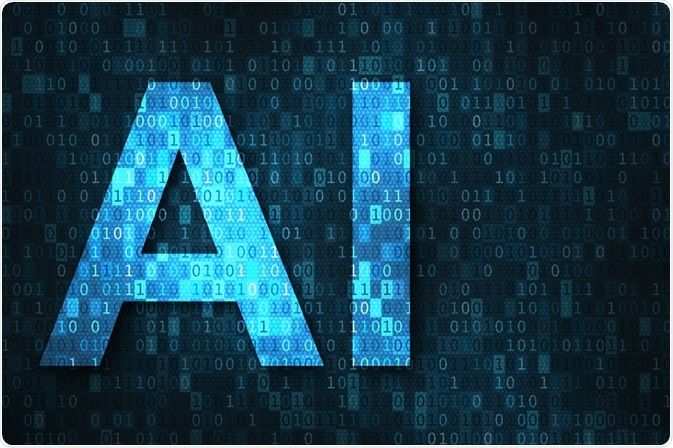
Image Credit: NicoElNino/Shutterstock.com
Consequently, artificial intelligence (AI) is helping in sorting, analyzing, and interpreting genomic data. Here, we take a closer look at what artificial intelligence is and how it is advancing the field of genomics.
What is AI?
AI is the simulation of intelligence in machines, allowing machines to perform tasks that would ordinarily need human intellect. In genomics, AI relies on machine learning, where algorithms spot patterns or classify inputted data within the dataset, applying what the computer system has learned to new data.
Primitive forms of machine learning require more extensive training in how to classify or recognize the data. But modern AI-based genomic approaches are now moving towards advanced machine learning algorithms, like deep learning.
Deep learning is sculpted more on how our brains pick out information. It is made from a series of artificial neural networks, often likened to the web of neurons in our brain. This artificial neural network is a hierarchy of layers, where each layer has data coming in, which is then transformed into new information (known as a feature) by mathematical functions.
These features can then be passed into different layers, which perform further data transformations, gaining more information and new features.
Deep learning automatically uses the features to spot patterns in the data and classify the information. Consequently, deep learning requires less input by humans than traditional AI and is used in complex genomic datasets.
AI in genomics: medical applications
By designing deep learning algorithms to compare genetic sequences, phenotypic outcomes can be predicted from genetic differences. And by analyzing differences between sequenced genomes, deep learning models can pick out diagnostic and drug targets and predict pathologies and prognoses of patients.
Consequently, many modern AI genomics systems are geared towards personalized medicine, where therapies are tailored to patient groups based on their genetic and environmental data, as well as their lifestyle.
AI is also improving the poor success rates seen in drug development — with only ~6% of drugs reaching the market. Using information from single studies and meta-study analyses, deep learning compares genome sequences and phenotypic information from different databases and literature sources, to better predict drug targets. These sequences can also be used in developing biomarkers for diagnostics.
For diseases caused by genetic abnormalities, gene-editing technology may be used to modify DNA sequences and alleviate symptoms, by using tools such as CRISPR-Cas9. Amongst the ethical considerations of gene-editing technology, it is also limited by the accuracy and sensitivity of off-site target effects.
To combat this, deep learning systems have been designed such as DeepCRISPR, which predicts on- and off-site sequence targets with high accuracy, whilst predicting phenotypic effects from genetic alterations. As CRISPR technology is widely used in biological and medical research, improving accuracy through AI will have many applications.
There is also growing interest in using AI to provide pharmacological insight, such as finding the optimal drug doses for different patient groups. By combining genomic data from patients along with drug mechanistic information, AI is being developed to understand the variability in drug response between different patients.
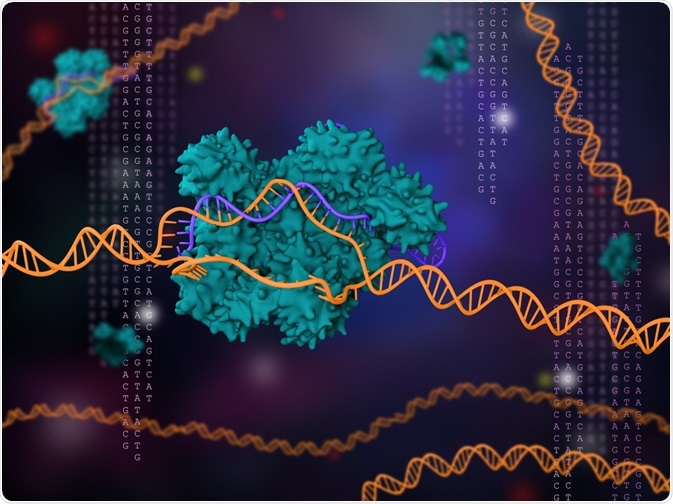
Image Credit: Meletios Verras/Shutterstock.com
AI in genomics: agricultural applications
AI is also pushing the capabilities of the field of genomics in agricultural applications. Traditionally, crop breeding programs are lengthy and costly, due to the complex interactions between genetic and environmental factors.
But with AI, scientists can design systems to manage these multiple, complex datasets, speeding up breeding programs.
Furthermore, these breeding programs can be used to develop disease-resistant crops. By using machine learning systems, scientists can predict disease-resistant genes, from analyzing multiple sources of data, to produce disease-resistant crops, such as rust-resistant wheat.
Conclusions
While a selection of applications has been discussed, the use of AI in genomics is varied and vast and will only continue to impact the field of genomics. Traditionally, AI has been used in genomics to speed up tasks that are vulnerable to error, the future of AI is moving towards a tool that also provides greater insight from multiple and complex datasets.
References
- Bini, S. A. (2018) ‘Artificial Intelligence, Machine Learning, Deep Learning, and Cognitive Computing: What Do These Terms Mean and How Will They Impact Health Care?’, Journal of Arthroplasty, 33(8), pp. 2358–2361.
- Chuai, G. et al. (2018) ‘DeepCRISPR: Optimized CRISPR guide RNA design by deep learning’, Genome Biology, 19(1), p. 80.
- Cutter, G. R., and Liu, Y. (2012) ‘Personalized medicine: The return of the house call?’, Neurology: Clinical Practice, 2(4), pp. 343–351.
- Dias, R. and Torkamani, A. (2019) ‘Artificial intelligence in clinical and genomic diagnostics’, Genome Medicine, 11(1), p. 70.
- Esposito, S. et al. (2020) ‘Applications and trends of machine learning in genomics and phenomics for next-generation breeding’, Plants, MDPI AG, 9(1).
- Hutchinson, L. et al. (2019) ‘Models and Machines: How Deep Learning Will Take Clinical Pharmacology to the Next Level’, CPT: Pharmacometrics and Systems Pharmacology, 8(3), pp. 131–134.
- Ornella, L. et al. (2017) ‘Applications of genomic selection in breeding wheat for rust resistance’, Methods in Molecular Biology, 1659, pp. 173–182.
- Vamathevan, J. et al. (2019) ‘Applications of machine learning in drug discovery and development’, Nature Reviews Drug Discovery, 18(6), pp. 463–477.
- Wong, C. H., Siah, K. W., and Lo, A. W. (2019) ‘Estimation of clinical trial success rates and related parameters’, Biostatistics, 20(2), pp. 273–286.