Within the fields of chemistry and the analytical chemistry subsection, scientists are charged with synthesizing compounds with effective moieties and functional groups that can regulate and inhibit disease. In the past, and holding true to this day, the turnover rate of efficacious compounds was found to be as low as 10%.
Analytical Chemistry" />
Image Credit: cono0430/Shutterstock.com
This means a 10% chance of clinical trials passing to stage two of the drug development cycle. However, this success rate is increasing with every passing day, as high throughput screening, supervised learning, and deep learning progress. This has allowed users to prioritize specified drug moieties through computational models, bypassing the need to screen all related compounds.
Not only does this save on valuable lab time and research working hours, it also addresses the fields of green chemistry and retains valuable resources. Through these in silico methodologies, we avoid an over-expenditure of reagents, lab kits, and supplementary costs.
The Various Iterations of Machine Learning
Designing target compounds and ushering in new remedies has been both a scientific practice and an art. Machine learning and its subsections of supervised learning, and deep learning, have become paramount in bringing drug discovery out of its slump. Machine learning, in essence, takes small data sets and categorizes/files them in a concise and organized manner that the user easily interprets. Supervised learning incorporates datasets to train algorithms, which classify data/ predict accurate outcomes. Finally, deep learning works with unstructured data and a surfeit of numbers, arranging the larger volumes into manageable bytes.
The operations of machine learning through a “business lens” often follow thusly: Organizations and labs such as eMolecule, Apollo Scientific, ChemDiv, and more will garner databanks worth of reaction pathways, possible drug moieties, and other data relating to analytical chemistry. This could be akin to organizations like Facebook or Enlyft, which collect user data (cookies). In turn, partnering organizations like Spaya, Creyon Bio, or BridGene Biosciences will gather that information and subject it to machine learning in the hopes of realizing a finalized product.
The Inner Workings of Machine Learning
The whole field of machine learning can be condensed to the interpolation of data via curve fitting. Curve fitting through high dimensional space is a practice that human beings are unable to accomplish, requiring a large amount of processing speed. However, it is important to note that this methodology does not produce an omniscient Blackbox and will not conceive the unknowable.
For the software and programs to work, they must abide by the rules of chemistry, meaning that they must decern between regioselectivity, chirality, and chemoselectivity. At the end of the day, it must set an arbitrary scoring rank to compare drugs to one another.
This is how desired reactions are discovered. This involves the collection of millions of reactions, cleaning said data (implying the removal of duplicates), and removing spectator compounds (redundant elements that exist in both the reactant and product side of a chemical reaction) solvents. This is followed by mapping specific reaction types and grouping them together to narrow down the final reaction types.
Outside the realm of retrosynthesis, machine learning may also be used to find the correct route in synthesizing carbohydrates and other macromolecules that may prove useful to the healthcare industry. These processes will require sublime stereocontrol and extensive protecting group strategies. However, the discovery process involved in this 3d space may prove challenging to all AI software, and in this case, a subject matter expert will be needed to curate the results.
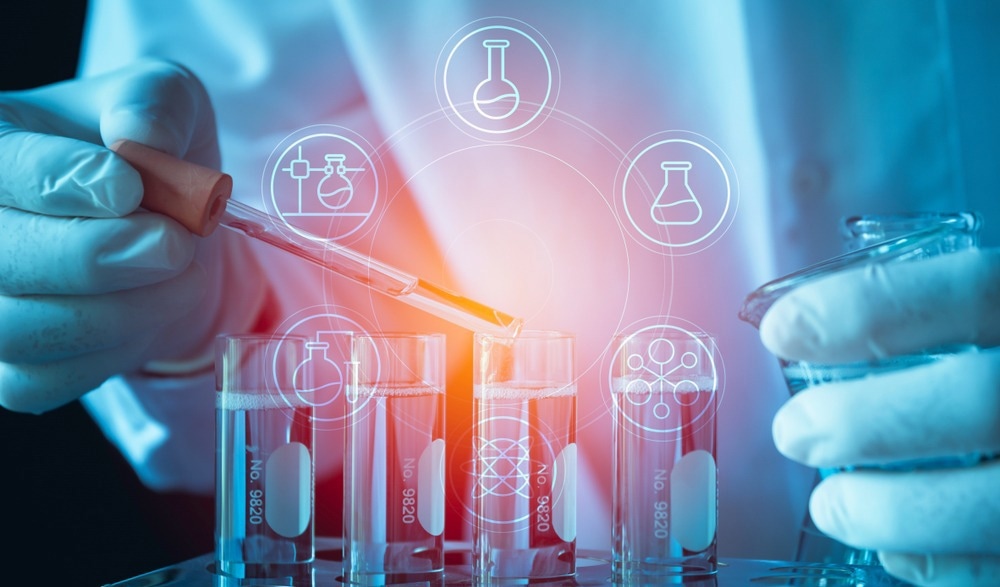
Image Credit: Krisana Antharith/Shutterstock.com
Supervised Learning and Deep Learning in Analytical Chemistry
Through the fine-tuning and processing of certain algorithms, the fields of proteomics, protein folding to be more specific, has progressed exponentially. A case study provided by the AlQurashi lab shows that AI methods are proven to be quicker when compared to AlphaFold software. However, AlphaFold is shown to be slightly more accurate when predicting protein structures when compared to reference structures.
However, even this flaw was ameliorated. This study predicted secondary protein structures using MATLAB through a feed-forward supervised learning mechanism (followed by a backpropagation error algorithm). The trained input and output data sets were evaluated, finding that the prediction of two-dimensional structures encompassed an accuracy of 62.72%.
Deep learning has been similarly used in mapping neuronal pathways, where extensive data sets of neurons are accrued, and simulations are performed to see how neurons are interconnected. The AI involved in these schematics “learn” through adjusting connection weights between nodes, taking input sets, and fitting them to output measurements.
Limitations of Machine Learning in Analytical Chemistry
In this broad field of analytical chemistry, machine learning cannot perform all the tasks we wish it to perform. Though it may seem hard to believe, there are some fields in which human beings perform better than machines. Machine learning can perform retrosynthesis to unearth a target molecule when constructing and deconstructing chemical reactions. However, if one wishes to design a total synthesis of natural products, where we tackle the start of a synthetic pathway to reach a product (rather than the converse), human intuition and spatial reasoning are still the preferred modus.
Human cognization largely trumps machine learning when assaying patterns, qualitative trends, and spatial reasoning. This, for example, is why you are asked to identify pictures or non-uniform letters and numbers of varying fonts when you are asked to “prove you are not a robot”.
Read Next: What is Quantum Biology?
Sources:
- Paul, D., Sanap, G., Shenoy, S., Kalyane, D., Kalia, K., & Tekade, R. K. (2021). Artificial intelligence in drug discovery and development. Drug discovery today, 26(1), 80–93. https://doi.org/10.1016/j.drudis.2020.10.010
- David H. Freedman. (2019) Hunting for New Drugs with AI. Nature. ISSN 1476-4687. doi: https://doi.org/10.1038/d41586-019-03846-0
- Zippel, C., & Bohnet-Joschko, S. (2021). Rise of Clinical Studies in the Field of Machine Learning: A Review of Data Registered in ClinicalTrials.gov. International journal of environmental research and public health, 18(10), 5072. https://doi.org/10.3390/ijerph18105072
- Zimányi L, Sipos Á, Sarlós F, Nagypál R, Groma GI. Machine-learning model selection and parameter estimation from kinetic data of complex first-order reaction systems. PLoS One. 2021 Aug 9;16(8):e0255675. doi: 10.1371/journal.pone.0255675. PMID: 34370771; PMCID: PMC8352076.
Further Reading