The last two decades have witnessed extraordinary advancements in the field of Artificial Intelligence (AI). Once a futuristic vision now turning into reality, AI is already changing the way people do science, with repercussions in everyday life.
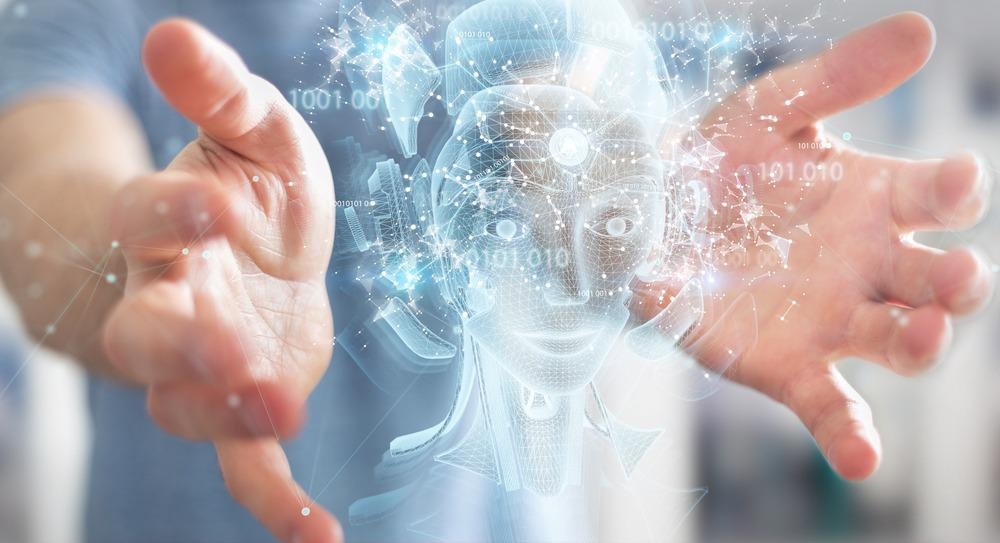
Image Credit: sdecoret/Shutterstock.com
AI is a fast developing branch of computer science that aims to imitate human behavior and conventional response patterns in order to perform human-like tasks. If properly programmed, AI enables machines to learn from experience and adjust to new inputs.
Generally, AI works in combination with machine learning and data analytics, processing different sorts of data, namely digital information, images, text, or unstructured data. The fields of application in daily life are countless, spanning from finance, transportation, healthcare, and security, among many others.
The technology behind smartphones and smart home devices is based on artificial intelligence (such as the renowned Google assistant and Alexa), while in finance, particular algorithms have been developed to find the best investment solutions.
Transportation is another sector where AI is producing major innovations, particularly concerning autonomous vehicles. Cars are equipped with cameras and sensors that help prevent collisions. Such devices provide a huge amount of information that needs high-performance computing for instant processing.
Similarly, navigation apps rely on GPS information and the analysis of real-time data from users to calculate the best route and minimize travel disruptions.
The Potential to Revolutionize Scientific Research
The capacity to analyze enormous amounts of data and to perform labor-intensive tasks in short times, makes AI extremely appealing, stimulating researchers to explore applications in several scientific fields.
Molecular biology could benefit considerably from AI. There is a lot of interest in trying to predict the three-dimensional structure of proteins from DNA sequences. Similarly, many efforts are being made in astronomy and the study of the universe.
Space observations in fact produce huge amounts of data where instrumental artifacts need to be identified and removed before real data can be analyzed. AI and machine learning can perform this challenging task, “cleaning” data for later analyses.
Transforming Medical Imaging and Clinical Diagnosis
There is an increasing interest in applying artificial intelligence techniques to medical imaging, particularly in the field of radionics, where a large number of quantitative features are extracted from medical images using data characterization algorithms.
AI can boost the healthcare sector by helping with the identification of tumors. Predictive models can be developed to identify regions of interest (ROI) or gross tumor volume (GTV) from images acquired via a scanner machine (e.g., MRI, CT-scan, PET).
Computers can be trained to distinguish between normal- and irregular-looking lymph nodes in computer tomography images, and a level of precision has been achieved in interpreting mammograms for breast cancer screening.
A cloud-based imaging platform (Arterys) that helps physicians track tumors on CT scans and MRI of lung and liver cancer patients, was approved by the food and drug administration (FDA) in 2018. While specialists can only analyze a limited number of images in their time, AI can contribute by performing multiple screenings and shortening analysis time, contributing to early diagnosis.
Artificial Intelligence for Disaster Management
Disaster management deals with large volumes of information, whether from real data or simulations. AI has the capacity to process such voluminous data and extract useful information to support effective decision-making in disaster management.
AI techniques can serve as an alternative in the hard task of real-time identification of upcoming disasters and issue of early warnings, which currently rely on experts’ analyses of sensor measurements in the field.
An example is the Urban-Flood project in Europe, where an internet-based platform for early flood warnings has been established. Based on the analysis of thousands of sensor streams, an AI component can detect abnormal dike behaviors.
One of the keys to efficient disaster response is the development of maps of the impact areas. AI methods can also generate event maps and damage information that can provide vital insights for the planning of search and rescue operations.
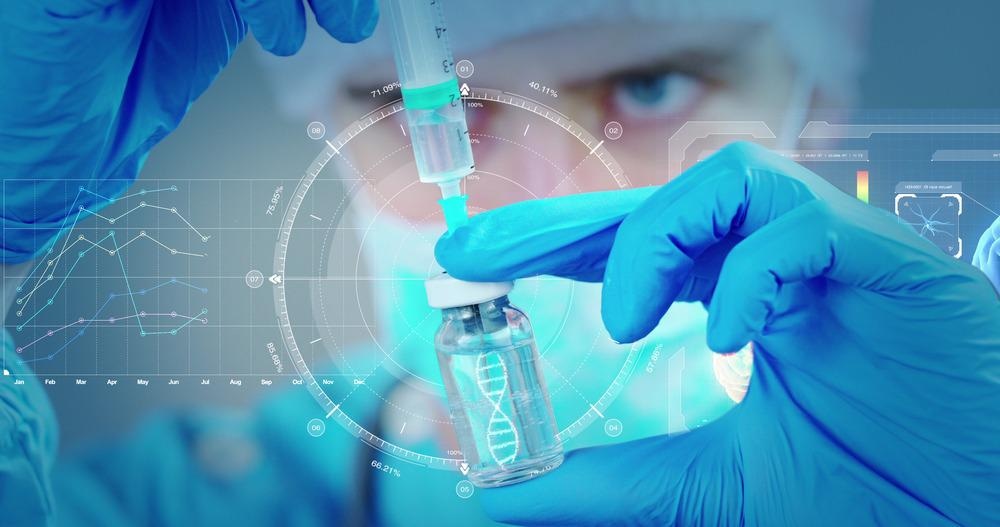
Image Credit: HQuality/Shutterstock.com
Can AI Change the Future of Chemical Synthesis?
There is a lot of interest in applying AI to medicinal chemistry for the identification and optimization of target molecules. Synthetic prediction tools can accelerate and reduce failures in the development phase for the synthesis of new compounds.
Finding the optimal or acceptable set of reaction conditions can require time-consuming empirical screening. AI has the potential to speed up the process and reduce considerably the financial burden associated with it.
Artificial intelligence and machine learning have been used for the synthetic planning of small molecules, with some companies already employing in silico methods into their overall approach to accessing target molecules.
Although in theory AI models can elaborate suitable reaction conditions if appropriately trained, in practice, such models are difficult to develop due to a lack of high-quality data.
The ultimate frontier in organic synthesis is the development of fully automated reaction platforms. Currently, research on automated synthesis is restricted to a relatively small set of reactions and it is mainly in the proof-of-concept phase. Such automated platforms still require considerable human setup and planning, but the process may become more efficient as predictive chemistry tools are developed.
With many applications already showing an impact in the real world, and many more in the development phase, the disruptive potential of AI is evident. Further research could advance the frontiers of science even further, and revolutionize multiple areas. At the pace new methods are developing, the possibilities are endless.
Sources:
- Roberts, S., Mcquillan, A., Reece, S. & Aigrain, S. (2013). Astrophysically robust systematics removal using variational inference: application to the first month of Kepler data. Monthly Notices of the Royal Astronomical Society, 435, 3639-3653.10.1093/mnras/stt1555
- Chaddad, A., Katib, Y. & Hassan, L. (2021). Future artificial intelligence tools and perspectives in medicine. Curr Opin Urol, 31, 371-377.10.1097/MOU.0000000000000884
- Sun, W., Bocchini, P. & Davison, B. D. (2020). Applications of artificial intelligence for disaster management. Natural Hazards, 103, 2631-2689.10.1007/s11069-020-04124-3
- Struble, T. J., Alvarez, J. C., Brown, S. P., Chytil, M., Cisar, J., Desjarlais, R. L., Engkvist, O., Frank, S. A., Greve, D. R., Griffin, D. J., Hou, X., Johannes, J. W., Kreatsoulas, C., Lahue, B., Mathea, M., Mogk, G., Nicolaou, C. A., Palmer, A. D., Price, D. J., Robinson, R. I., Salentin, S., Xing, L., Jaakkola, T., Green, W. H., Barzilay, R., Coley, C. W. & Jensen, K. F. (2020). Current and Future Roles of Artificial Intelligence in Medicinal Chemistry Synthesis. J Med Chem, 63, 8667-8682.10.1021/acs.jmedchem.9b02120
Further Reading