The global population is expected to reach nine billion by 2050, necessitating a significant increase in agricultural productivity to meet rising food demands. However, challenges such as declining soil quality and limited arable land threaten the expansion of agriculture. Fortunately, emerging technologies offer solutions to boost agricultural productivity.
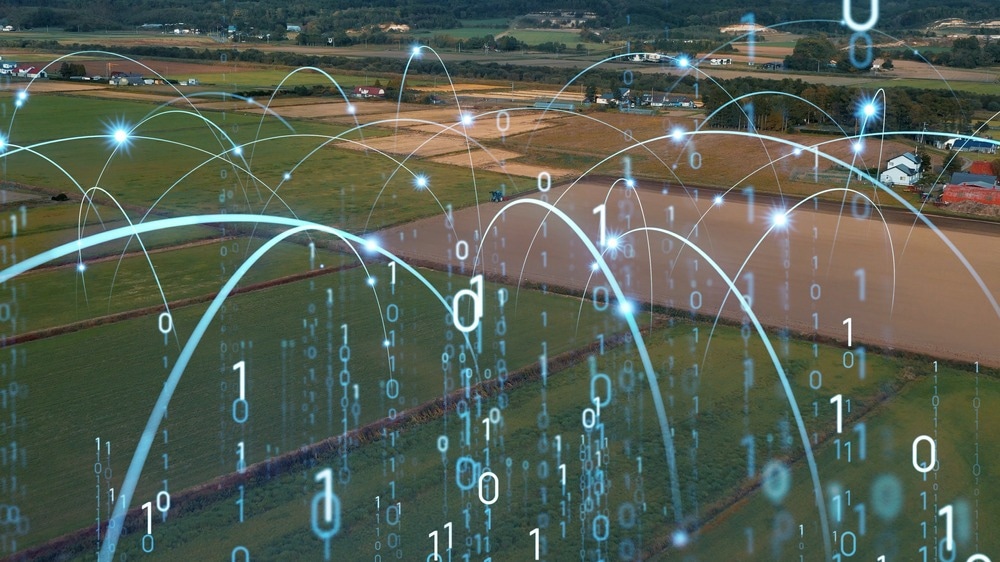
Image Credit: metamorworks/Shutterstock.com
The Digital Revolution and Big Data in Agriculture
In recent decades, the digital revolution, fueled by advancements in Information and Communication Technologies (ICT), has brought about significant innovations in various sectors, including agriculture. Big data has transformed how information is collected, stored, and utilized within ICT, enabling greater productivity and profitability in agriculture.
Big data refers to vast and varied data sets generated at high speed, characterized by the "3 Vs"—variety, volume, and velocity. Traditional data systems cannot handle these large datasets. Big data is particularly important in agriculture, where information can be collected and analyzed across different spatial and temporal scales using various platforms.
For agriculture, processing large amounts of data—from individual farm plots to satellite-derived environmental data—is essential for making informed management decisions. This is especially crucial in a rapidly changing world where environmental conditions and socioeconomic factors increasingly impact food production.
Advancements in Big Data for Agriculture
Himesh et al. (2018) reviewed the potential of big data to transform agriculture. Their study highlighted the use of big data throughout the food production process, from farm to food, noting that the ongoing digital revolution is likely to permanently change how food production is designed, managed, and executed.
Lytos et al. (2020) echoed these findings, referring to "agriculture intelligence" as the application of big data and intelligent technologies in the sector. Their research demonstrated how these technologies enable unprecedented capabilities for data collection and analysis across agricultural systems. The study concluded by addressing the knowledge gap between big data systems and their applications in agriculture, emphasizing the need for further development.
Learn more about agricultural science
Applications of Big Data in Agriculture
Literature reviews have demonstrated that big data can rapidly, accurately, and cost-effectively inform stakeholders about food productivity. The primary goal of agriculture is to maximize land use while increasing financial profitability, both of which can be enhanced through effective data collection and usage.
One notable application of big data is Geographic Information Systems (GIS), which allow researchers to create dynamic maps based on various types of information (e.g., environmental, social). The trend of using Big GIS Analytics (BGA) in agriculture has gained popularity in recent years. Sharma et al. (2018) designed a BGA framework to improve GIS applications in agriculture, further advancing this field.
Precision agriculture, which began in the late 1990s with the integration of GPS sensors into farm machinery, also benefits from big data. The Internet of Things (IoT) has accelerated the shift toward precision farming, enabling wireless networks and online resources to enhance agricultural management. Precision agriculture relies heavily on big data, as modern technologies require the processing of vast and diverse data sets to function effectively.
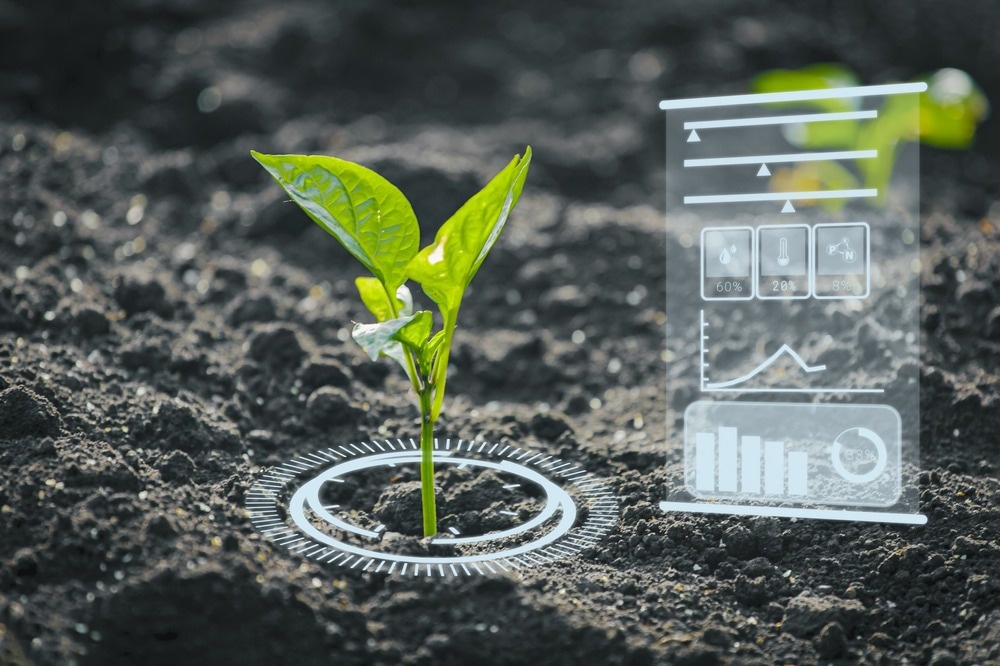
Image Credit: Leonid Sorokin/Shutterstock.com
Challenges in Adopting Big Data Analytics in Agriculture
Despite its potential, several challenges hinder the integration of big data analytics in agriculture. Emerging technologies aim to produce more food on less land, with fewer inputs and a reduced ecological footprint. However, barriers such as the complexity of data interpretation limit their effectiveness.
Weersink et al. (2018) reviewed the challenges of using big data in agriculture, noting that not all collected data is useful for decision-making. For example, certain climatic variables may not be relevant for predicting crop health, requiring a selection process to eliminate unnecessary data. Additionally, many farmers may lack the skills to interpret or utilize these tools, highlighting the need for education and training.
The authors emphasized the need for stakeholders, particularly farmers, to be equipped with the knowledge, tools, and financial resources to implement big data-driven decisions. By doing so, agricultural practices can better prioritize environmental concerns, monitor changes over time, and move toward more sustainable production methods.
Sources:
- Himesh, S., Rao, E. V. S. P., Gouda, K. C., Ramesh, K. V., Rakesh, V., Mohapatra, G. N., Rao, B. K., Sahoo, S. K., & Ajilesh, P. (2018). Digital revolution and Big Data: a new revolution in agriculture. CABI Reviews, 2018, 1–7. https://doi.org/10.1079/pavsnnr201813021
- Lytos, A., Lagkas, T., Sarigiannidis, P., Zervakis, M., & Livanos, G. (2020). Towards smart farming: Systems, frameworks and exploitation of multiple sources. Computer Networks, 172, 107147. https://doi.org/10.1016/j.comnet.2020.107147
- Misra, N. N., Dixit, Y., Al-Mallahi, A., Bhullar, M. S., Upadhyay, R., & Martynenko, A. (2022). IoT, Big Data, and Artificial Intelligence in Agriculture and Food Industry. IEEE Internet of Things Journal, 9(9), 6305–6324. https://doi.org/10.1109/jiot.2020.2998584
- Sharma, R., Kamble, S. S., & Gunasekaran, A. (2018). Big GIS analytics framework for agriculture supply chains: A literature review identifying the current trends and future perspectives. Computers and Electronics in Agriculture, 155, 103–120. https://doi.org/10.1016/j.compag.2018.10.001
- Weersink, A., Fraser, E., Pannell, D., Duncan, E., & Rotz, S. (2018). Opportunities and Challenges for Big Data in Agricultural and Environmental Analysis. Annual Review of Resource Economics, 10(1), 19–37. https://doi.org/10.1146/annurev-resource-100516-053654
Further Reading