Crop yield is a key driver of agricultural productivity. Methods predicting crop yield have transitioned towards using automated ‘intelligent’ techniques, such as machine learning computational models as well as vegetation indices. The accuracy of these methods is being improved continuously, ultimately providing essential information for agricultural policies and food security.
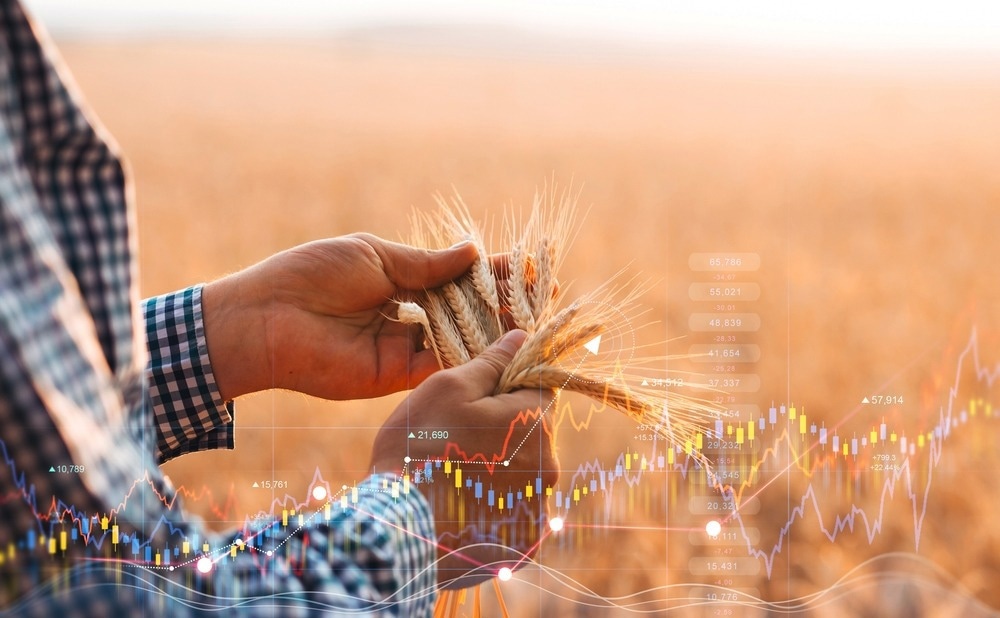
Image Credit: Miha Creative/Shutterstock.com
How to accurately predict crop yield
In a rapidly changing world, accurately predicting crop yield levels is central to designing effective agricultural strategies and maintaining food security. From policymakers to farmers and consumers, crop yield drives food productivity and plays a significant role in ensuring food safety and availability. Predicting crop yield is, therefore, a significant benefit to informing management and financial decisions.
Crop yield refers to the ratio between agricultural input and output and can be defined as the degree of yield per unit area of cultivated land. Therefore, crop yield is often used as an indicator of agricultural productivity. Crop yield can be affected by production measures, pests and pathogens, environmental changes, and consumer demand, among other factors.
As a result, crop yield monitoring is pivotal to every country’s agricultural and economic development. This is because crop yield can then inform import/export decisions as well as price structure, crop distribution, and future crop planning. Researchers have developed different ways to track and predict crop yield, including vegetation indices and statistical models.
The different ways of predicting crop yield were reviewed by He et al. in 2022 in a study focused on fruit harvesting data over the last decade. The authors highlight the transition towards automatic yield monitoring systems and away from time-consuming manual methods. The study discusses the range of vegetation indices that can be used across fruit types, environments, and data types and how machine learning models are becoming increasingly popular due to their capacity to increase prediction accuracy.
Machine learning can be used to refine the predictive power and accuracy of crop yield models. Statistical models extrapolating predictions based on available data have become widely used due to the availability of time series data such as weather variables or soil health. Many agriculture models use qualitative and quantitative data to improve yield predictions using intelligent computational techniques.
Limitations to predicting crop yield and their solutions
Whether traditional or modern, each method of predicting crop yield is associated with inherent limitations. In turn, this can have knock-on effects to affect overall crop productivity. This was discussed in the study by He et al., who discussed how complex natural environments with inconsistent horticultural management practices could pose significant challenges to crop yield estimations.
The use of vegetation indices using spectral data can be limited by context-specific information since growth rate changes across different orchard types can affect estimates of large-scale production. This can be solved by selecting a species-specific period to determine the growth status of fruit trees to obtain more accurate data. Although this may be more data-dependent since it requires information on each fruit tree species, this method has proved effective across orchard types, as seen in a 2012 study by Laurent et al.
For methods relying on computational modeling, model accuracy relies on stable trends in past data to infer future yield estimates, which can be confounded by unexpected changes in climate, soil, irrigation, or cultivation. The interactive effects of environmental conditions and changes in field management practices can hinder the accuracy of models. To address such limitations, He et al. (2022) suggest fusing different inference algorithms to obtain more accurate fits to variable data.
The choice of method to predict crop yield plays a key part in optimizing crop yield predictive accuracy. Methods of production based on large spatial or temporal scales may be able to use data derived from remote sensing technology, whereas smaller-scale practices may rely more on vegetation indices obtained from samples gathered from tractors, sub-sampled areas, or smartphones. In turn, each method can be optimized to improve accuracy over time by integrating different data types and using automated tools that can be used reliably and cost-effectively.
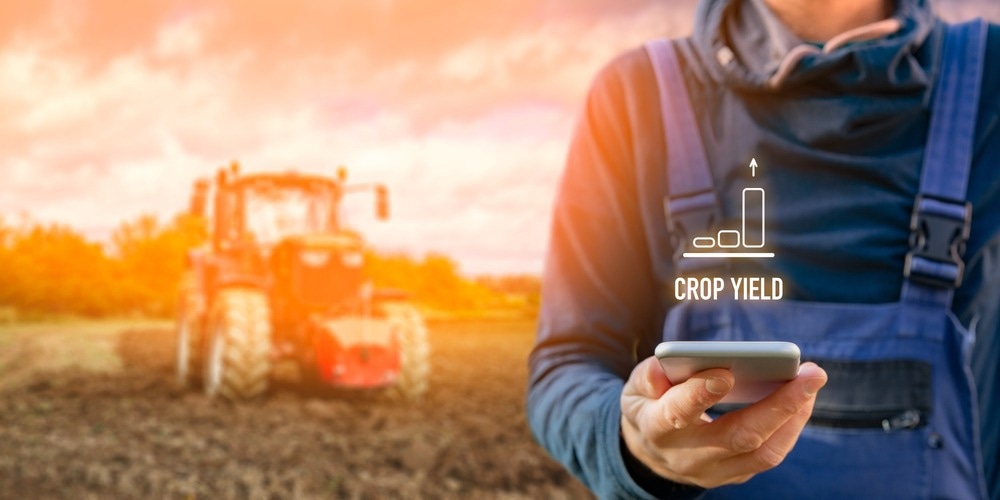
Image Credit: Jirsak/Shutterstock.com
Emerging techniques for predicting crop yield
Using a variety of tools can provide multidimensional and multifaceted data to input into computational models and vegetation indices, which remain the two most popular methods of predicting crop yield, according to the He et al. (2022) study. In recent years, several methods have been developed that integrate technological tools to improve the accuracy of crop yield predictions. For instance, using machine vision systems with image spectra or remote sensing technology to improve image processing has proved helpful for deep learning algorithms.
Technologies such as unmanned aerial vehicles collected (UAVs) were also used by Zeng et al. in a proof-of-concept study in 2021. The researchers used UAVs with red–green–blue (RGB) images to predict crop yield according to fluorescent images. Drone technology is becoming increasingly cost-efficient and accurate and integrating different image processing abilities, which can benefit farmers in estimating crop growth, development, health, and yield.
In turn, data from different technologies can inform complex computational models that are continuously improving, as Nagini et al. (2016) discussed. Other models are also being developed, including root-mean-square-error (RMSE) that use deep neural networks based on weather data to predict yield. Ultimately, researchers are continuously refining methods to estimate crop yield, which can be used to inform stakeholders, policymakers, and consumers, to enhance productivity and ensure food security.
Sources:
- He, L., Fang, W., Zhao, G., Wu, Z., Fu, L., Li, R., Majeed, Y., & Dhupia, J. (2022, April). Fruit yield prediction and estimation in orchards: A state-of-the-art comprehensive review for both direct and indirect methods. Computers and Electronics in Agriculture, 195, 106812. https://doi.org/10.1016/j.compag.2022.106812
- Khaki, S., & Wang, L. (2019, May 22). Crop Yield Prediction Using Deep Neural Networks. Frontiers in Plant Science, 10. https://doi.org/10.3389/fpls.2019.00621
- Laurent, C., Oger, B., Taylor, J. A., Scholasch, T., Metay, A., & Tisseyre, B. (2021, October). A review of the issues, methods and perspectives for yield estimation, prediction and forecasting in viticulture. European Journal of Agronomy, 130, 126339. https://doi.org/10.1016/j.eja.2021.126339
- Nagini, S., Kanth, T. R., & Kiranmayee, B. (2016, December). Agriculture yield prediction using predictive analytic techniques. 2016 2nd International Conference on Contemporary Computing and Informatics (IC3I). https://doi.org/10.1109/ic3i.2016.7918789
- Pant, J., Pant, R., Kumar Singh, M., Pratap Singh, D., & Pant, H. (2021). Analysis of agricultural crop yield prediction using statistical techniques of machine learning. Materials Today: Proceedings, 46, 10922–10926. https://doi.org/10.1016/j.matpr.2021.01.948
- Zeng, L., Peng, G., Meng, R., Man, J., Li, W., Xu, B., Lv, Z., & Sun, R. (2021, July 26). Wheat Yield Prediction Based on Unmanned Aerial Vehicles-Collected Red–Green–Blue Imagery. Remote Sensing, 13(15), 2937. https://doi.org/10.3390/rs13152937
Further Reading