Most individuals are keenly aware of the new rises in automation that parallel the advancements that we are making in artificial intelligence, machine learning, and dynamic processing. Just some of these areas include home care robots, tumor detection via image analysis, cardiovascular risk prediction, and much more. However, these trends may not be as linear and as simple as most make them out to be.
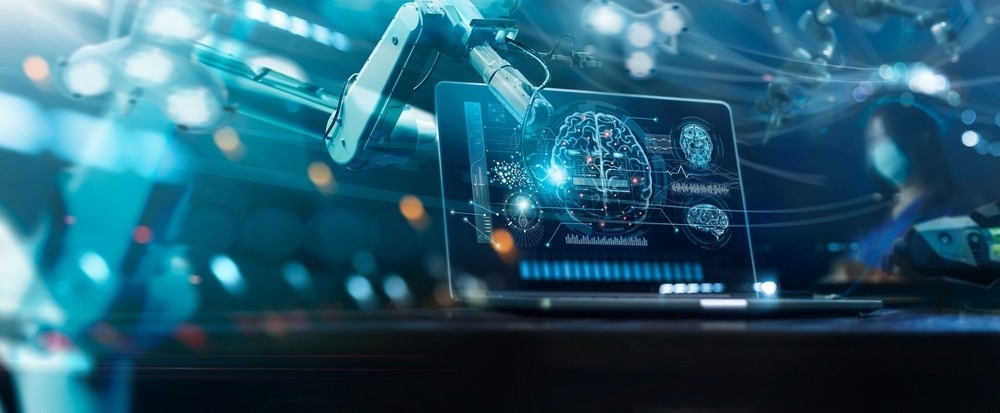
Image Credit: PopTika/Shutterstock.com
Worries about automated beings replacing humans must be considered, different classifications of robotics/automation must be proposed, and the question of what extent scientists should rely on AI must be addressed.
Addressing the Myths of Automation in Life Sciences
Protocols and applications present in academic life science labs rely quite heavily on machines. However, the fear that humans will be replaced with instruments throughout the workplace is somewhat misguided and highly exaggerated.
As new technologies develop, those who fund research proposals, survey operations, and lead innovations hold a benevolent culture of investing in people rather than machines. It is the task of the principal investigator, as well as the undergraduates, postgraduates, post-docs, and technical staff that are under him, to maintain and upkeep these automated processes we so highly rely on. In addition, this new frontier of automation will also further research programs and strengthen the ties between engineers, biologists, and chemists as novel research questions arise.
Artificial Intelligence Implemented Today
When discussing the general trends of robotics in life sciences, it is important to factor in physical equipment that can move objects in the physical world, as well as smart technologies and software-based systems (these can include data mining tools, image analysis software, etc.).
People often associate modern robotics with the coming of artificial intelligence (AI), a term applied to technology that mirrors critical thinking and amassing intelligence- akin to a human. This recent technological feat has improved the decision-making of healthcare professionals in diagnostics, monitoring patients’ physicality, surgery planning, and much more.
One area that is predominantly being affected by this coming-of-age technology is tumor detection. These technologies act as advisors to medical professionals. To accommodate the massive variability in tumor locations, we possess many tools to survey said tumors, such as MRI, PET, CT, X-rays, and more. AI can differentiate between unsuitable and appropriate scanning measures by improving the recognition of masses in the chest. Studies performed by Nam JG et al. (2020) and Andre Esteva et al. (2017) demonstrate that deep learning convolutional neural networks (CNN) can perform tumor localizations with greater precision than specialists.
The former study shows that unaided and aided radiologists performed slightly worse than AI algorithms when assaying lung cancer, with P values of less than 0.001. The later study exhibited a comparative assay between two dermatologists (with 65.6% and 66.0% accuracy) and two CNNs (with 69.4% and 72.1% accuracy).
A Structured Method to Assess Robotics
Though no all-encompassing classification has been proposed for the varying types of automation, an outline proposed by Frohm et al. (2008) introduces a tier list that catalogs industrial forms of automation on account of their complexity, cost, and required manpower. These automation levels include the following:
- Completely manual- requiring no tools, moving solely through muscle power
- Static hand tools- physical instruments that require a user (hammer, wrench, etc.)
- Flexible hand tool- manual work accompanied by a flexible tool (adjustable spanner, sextant)
- Automated hand tool- human hands which operate a mechanical apparatus with moving parts (power drill)
- Static Machine/workstation- machine automated work that is directed to one specific function, without any “deep learning elements” (lathe)
- Flexible machine/workstation- an automatic machine that can work multiple different tasks (CNC machine
- Totally automatic machine- An autonomous machine that will solve internal malfunctions, and deviations without the assistance of humans (mars rover)
This classification system has aided new benchtop chemists in understanding the different factions of automation. While traditional wet chemistry and biology labs typically contain automation levels of 5 or 6, the “second machine age” we now live in sees an exponential trend in mechanic potential and an increased presence in higher automation levels.
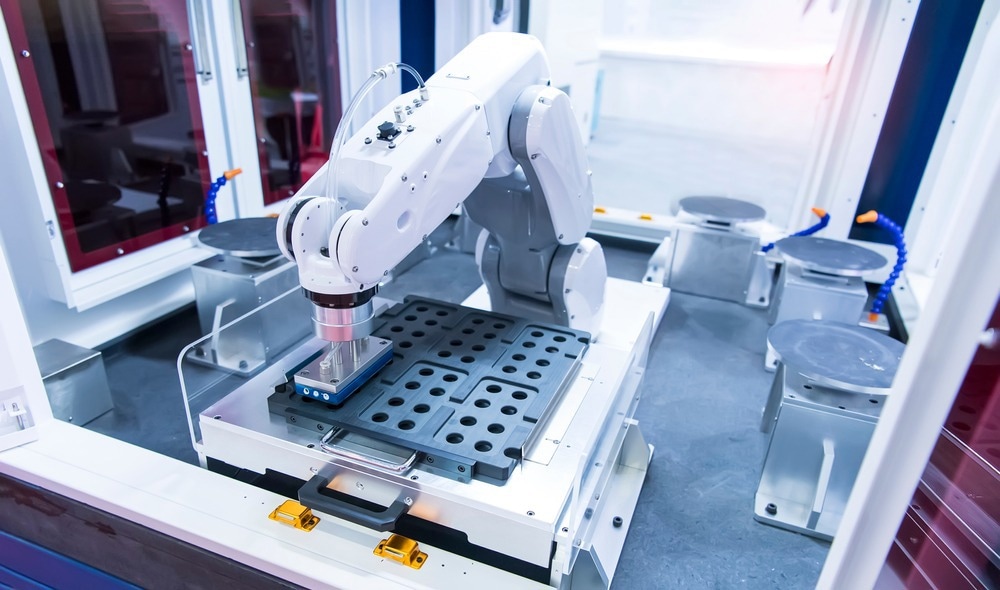
Image Credit: asharkyu/Shutterstock.com
The Robotics Used in Laboratories
Labs such as the Lilly Life Sciences Studio labs, powered by Strateos, Inc., are using silicon-based robotics coupled with cloud lab processes executing thousands of experiments a day, with very limited human intervention (falling under the 6th automation level). Currently, we see human beings doing very labor-intensive work: manually pipetting, manually placing vials in and out of centrifuges, and moving plates in and out of incubators.
However, by designing code that can be implemented within these silicon-based devices, a great deal of work can be offloaded by humans while at the same time showing massive improvements in repeatability and reliability.
If a principal investigator was to offer a protocol to an understudy of his, ambiguous steps could often be found, which are interpretable in many ways. For example, a lab technician can be asked to shake a vial until it is cloudy or presents a certain hue or translucence. The amount of time one should shake, and the optic density presented within the vial, are very open to interpretation, and small changes such as this can drastically affect the result of an experiment.
Another common task asked of workers in wet labs is to incubate overnight or let a certain species/ solution sit in a cold room within a range of times. By determining the set parameters in code, these issues of vagueness are circumvented, and adjustments to the protocol can, in turn, be made.
Sources:
- Ian Holland, Jamie A. Davies et al. (2020) Automation in the Life Science Research Laboratory. Deanery of Biomedical Science and Synthsys Centre for Synthetic and Systems Biology University of Edinburgh https://doi.org/10.3389/fbioe.2020.571777
- Frohm J., Lindström V., Winroth M., Stahre J. (2008). Levels of Automation in Manufacturing. Int. J. Ergon. Hum. Fact. 30 71–74.
- Frohm, Jörgen. (2008). Levels of automation in production systems. 10.13140/RG.2.1.2797.7447.
- Abramo G., D’Angelo C. (2014). How do you define and measure research productivity? Scientometrics 101 1129–1144. 10.1007/s11192-014-1269-8
- Gao W, Raghavan P, Coley CW. (2022) Autonomous platforms for data-driven organic synthesis. Nat Commun;13(1):1075. doi: 10.1038/s41467-022-28736-4. PMID: 35228543; PMCID: PMC8885738.
- Coley CW, et al. (2019) A robotic platform for flow synthesis of organic compounds informed by AI planning. Science;365:eaax1566. doi: 10.1126/science.aax1566
- Mikulak-Klucznik, B. et al. (2020) Computational planning of the synthesis of complex natural products. Nature2020, 1–11. 10.1038/s41586-020-2855-y
Further Reading