Bioinformatics is defined as the mathematical interpretation of biological data and frequently utilizes computational methods to provide statistical information.
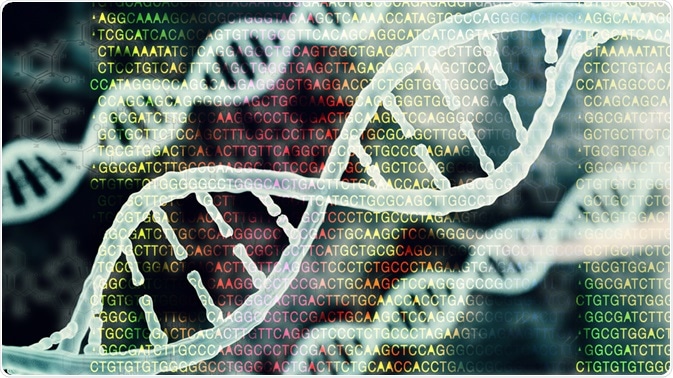
Bioinformatics. Image Credit: CI Photos/Shutterstock.com
Machine learning is a thriving field of computer science that entails the creation of algorithms that allow for the incorporation of new data to improve or develop the actions involved in a particular task.
One example of an application of machine learning includes e-mail filters that are able to learn which e-mails are likely to be considered as junk by the user. Correspondingly, the large quantities of data that must be handled in biology (particularly genomics and proteomics) mean that the field is well disposed to the application of machine learning.
How is machine learning currently used in bioinformatics?
Machine learning is currently employed in genomic sequencing, the determination of protein structure, microarray examination, evolutionary phylogenetic tree construction, as well as metabolic pathway determination, among others.
The very large amount of genetic sequence information generated in the past several decades has provided massive data banks that defy the ability of human researchers to effectively examine and process this information without the aid of computational methods.
Gene prediction is performed by machine learning algorithms in a number of ways - including inputting large quantities of DNA sequences that are compared with known libraries of genes and their locations noted.
Unrecognized genes in the sequence are identified by machine learning programs that predict their function based on the locus of the gene, among other factors. Finally, the comparison of the genomes of many different species is used to determine evolutionary trees.
Protein structure is predicted by machine learning programs by analyzing the amino acid sequence. The number of possible structures for proteins with identical amino acid sequences is huge, and thus the many thousands of possible confirmations are best analyzed using computational methods.
This may be done in a variety of ways, though among the most common is the sequential simulation of each conformation and the analysis of the surface energy profile of each in order to determine the most likely energetically favorable structure.
What does the future look like for Machine Learning?
Other fields within medicine and biology are increasingly falling under the purview of machine learning applications, as the technology becomes ever more sophisticated. For example, images created by neuroimaging techniques such as CT and MRI are now being analyzed by machine learning programs with the hope that scientists can gain insights into early disease symptoms and characteristics. This is particularly useful for brain and cardiac disorders, as the programs can search through and compare many thousands of results to find commonalities between them.
Any field in which large bodies of data are generated that can be compared with one another are suitable for machine learning applications, including both text and image data mining. Machine learning programs will be used increasingly for both research purposes and clinical applications.
Interesting and potentially significant conclusions drawn from machine learning algorithms may be highlighted for researchers to investigate more thoroughly, while such programs have been shown to analyze images with similar or greater success than humans.
The largest hurdle to machine learning in the future is not the availability of large quantities of data, but the computing resources available for such programs. Additionally, machine learning algorithms must still be checked for validity by human operators, which often represents a more time-consuming process than the analysis performed by the computer.
Further Reading