The incorporation of bioinformatics into the drug discovery and development industry is often referred to as translational bioinformatics, which was recognized and subsequently defined by the American Medical Informatics Association (AMIA) in 2006.
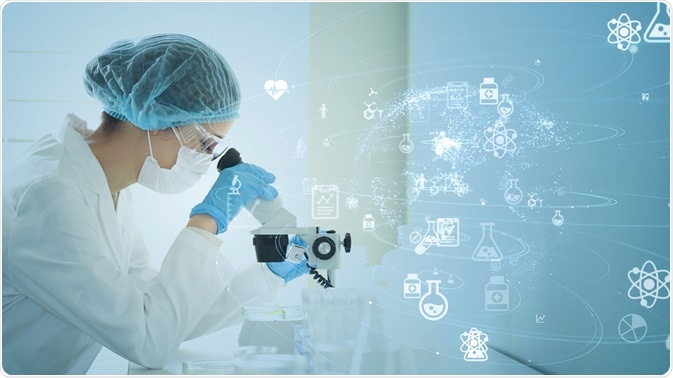
Drug Discovery. Image Credit: metamorworks/Shutterstock.com
Herein, AMIA defined translational bioinformatics as the development of storage, analytical, and interpretive methods that, taken together, can increase the availability of both biomedical and genomic data for novel drug discoveries.
Translational bioinformatics in action
Despite the medical advancements and discoveries that have revolutionized how diseases are diagnosed and treated over the past several decades, researchers estimate that only approximately 30% of all known diseases can be treated with currently available pharmacological agents. This gap in the availability of tested and approved therapies is further expanded by the fact that many biological targets have yet to be identified for many communicable and non-communicable diseases.
In addition to a lack of treatment options for known diseases, the emergence of novel viruses like the severe acute respiratory syndrome coronavirus 2 (SARS-CoV-2) further emphasizes the need to improve the rational drug design process.
Advancing the tools available for translational bioinformatics is one potential resolution to this growing problem. With its incorporation into almost every phase of the drug discovery process beginning with preclinical research on novel drugs and continuing throughout clinical trials and post-launch, translational bioinformatics can undoubtedly assist in the discovery of effective drugs for both communicable and non-communicable diseases.
Genome sequencing and drug discovery
Originally launched in 2003, the Human Genome Project (HGP) emerged with their initial discovery of 20,000 to 25,000 protein-coding genes that were believed to make up approximately 1.5% of the total human genome. Since then, several additional projects have emerged intending to identify all functional elements of the human genome, including any variations that might contribute to specific diseases.
Specific applications including gene sequencing, genetic statistics, and the measurement of gene expression levels which, taken together, have improved the dose-response, toxicity profile, and overall efficacy of drugs that are used to treat many different genetic diseases.
Despite these advantages, there remains a lack of certainty surrounding the functional range of many of the identified human genes. To further advance the identification of disease-linked genetic anomalies, bioinformatics researchers have now shifted their efforts towards characterizing and measuring gene expression levels of functional genes.
Fortunately, several databases are currently available to meet these demands. Human genome sequencing programs, for example, have collectively provided information to the public on over 17 million human single nucleotide polymorphisms (SNPs). While this library of genetic data is impressive, it is associated with several limitations as a result of its massive size that limits its usefulness for drug discovery purposes.
Comparatively, the Online Mendelian Inheritance in Man (OMIM) database, which provides a collection of human genetic variations and their potential link to all known Mendelian disorders. This type of platform not only allows researchers to have a better understanding of the potential domino effect that a single genetic mutation can have but also provides direct links to applicable publications for the user’s convenience.
Comparative genome analysis
Comparative genomic analysis, which involves the comparison of genome sequences from multiple organisms, is a powerful tool that is widely used during the design process of novel drugs.
When investigating potential therapeutics for infectious diseases, researchers can utilize this bioinformatics technique to identify gene targets that might be present within a given pathogen but are absent in its host. Some of the specific genetic variations that are studied in comparative genome analysis include both nucleotide and amino acid sequence alignments.
One particularly useful rapid search algorithm that has been developed to assist in the identification of potentially orthologous genes and/or related proteins includes the Basic Local Alignment Search Tool (BLAST). The BLAST program utilizes a pairwise approach in which local or global alignments between only two sequences at a given time are identified upon the search of a single sequence.
BLAST provides the investigator with both an alignment score, which measures the extent of the local ungapped alignment, as well as an e-value, which represents the statistically significant threshold for the reporting sequence matches against individual genomes.
Phylogenetic methods
As compared to similarity-based methods like BLAST, the ClustalW, and FAST Alignment (FASTA) methods, phylogenetic methods are considered to be superior in their ability to consider the potential effects of repeated substitutions at one site.
Furthermore, phylogenetic trees are used to reconstruct evolutionary networks among related organisms to allow for the analysis of any significant genealogical relationships. Some of the most popular web-based tools that allow users to construct and analyze phylogenetic trees include Phylogeny.fr, ClustalW2-phylogeny, PhyloT, iTOL, NCBI Tree Viewer, and T-rex.
For drug discovery purposes, phylogenetic trees can allow clinicians to reconstruct the history of an individual’s disease, particularly cancer and infectious diseases, to determine whether a given therapy will help or hurt the patient.
Repurposing approved drugs
In addition to creating more patient-centered treatment approaches, translational bioinformatics methods have also been used to reposition drugs that have already been approved by the United States Food and Drug Administration (FDA) to treat one type of disease for the treatment of another type.
The repositioning of available drugs for treating conditions that differ from its original treatment purposes has been shown to significantly reduce the costs and time requirements that are typically associated with the early stages of the drug discovery process. Some of the different bioinformatics methods that have been used for this purpose include the analysis of transcriptomic data for drug-disease relationships, meta-analyses of genomic data and drugs, the discovery of redundant molecular pathways, and the profiling microarray data sets.
By fast-tracking the drug discovery process, researchers can not only provide immediate relief to patients but also reduce rising healthcare expenses. Patients with autoimmune diseases like inflammatory bowel disease (IBD) have already benefited from such approaches, as gene-level profiling of IBD subtypes and their associations with other diseases has been used to discover potential drug candidates that can be used for repositioning.
Conclusion
Taken together, many different predictive bioinformatic approaches are currently available for public use. Although each of these tools is associated with its advantages and weaknesses, bioinformatics remains one of the leading scientific domains that is currently used in the drug design process.
References and Further Reading
- Buchan, N. S., Rajpal, D. K., Webster, Y., et al. (2011). The role of translational bioinformatics in drug discovery. Drug Discovery Today 16(9-10); 426-434. doi:10.1016/j.drudis.2011.03.002.
- Ramharack, P., & Soliman, M. E. S (2018). Bioinformatics-based tools in drug discovery: the cartography from single gene to integrative biological networks. Drug Discovery Today 23(9); 1658-1665. doi:10.1016/j.drudis.2018.05.041.
- Yan, Q. (2017). Chapter Eight – Translational Bioinformatics Methods for Drug Discovery and Development. Translational Bioinformatics and Systems Biology Methods for Personalized Medicine; 97-110. doi:10.1016/B978-0-12-804328-8.00008-5.
Further Reading