Modern fisheries are experiencing rapid changes due to the modification of environmental conditions as well as an intensification of fishing efforts. In response, computational models are being used to understand these changes and to better address their impacts.
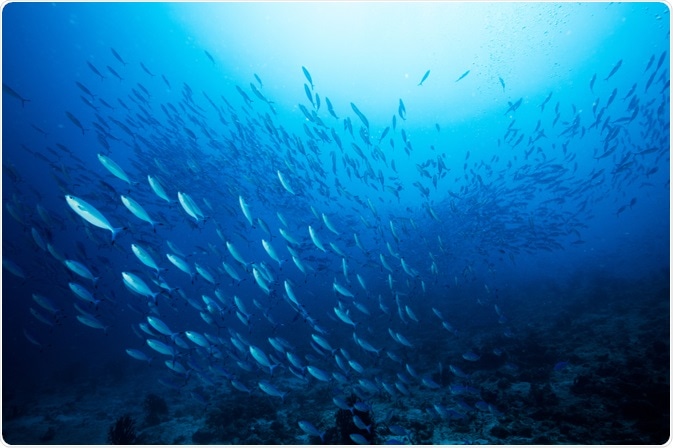
Fish. Image Credit: D.Lertchairit/Shutterstock.com
The implications of using computational models in fisheries
The growing demand for aquatic resources as well as the emergence of more efficient technology adapted to detecting, catching, and producing such resources, has resulted in widespread effects across fisheries.
For instance, exploited fish populations have experienced genomic changes as well as altered life-history traits and phenotypic characteristics. In turn, such changes are predicted to impact human populations.
In a 2019 study led by Lauric Thiault, researchers explored the ‘perfect storm’ of climatic and anthropogenic stressors occurring across fisheries. The predictive models indicated that under a business-as-usual future, nearly 90% of the human population will be exposed to losses of food production by 2100 as a result of a decline in agricultural and fisheries productivity.
Such findings highlight the potential impacts of environmental change currently occurring in fisheries as well as the value of using computational models to understand why and how practices could be improved.
Computational modeling refers to the use of computational science to study mathematical equations of interest by adjusting parameters of the system to examine potential differences in the outcome of each model. Therefore, models have become akin to other processes of scientific experimentation as hypotheses can be designed and disproven by developing relevant models.
Consequently, modeling has been applied to many disciplines including fisheries science to improve the identification, monitoring, and prediction of changes in fisheries stocks into the future.
Fisheries as ‘model’ systems for computational modeling
Fish stocks provide some of the longest monitoring of natural resources in human history, with the oldest trade of dry cod from Norway to southern Europe, which started at least 1000 years ago during the Viking era, still ongoing to this day.
In 1883, the Marine Biological Association was founded in Plymouth, UK, to essentially resolve the issue of whether fish stocks were limitless. Today, such an argument would be insubstantial due to the well-documented records and extensive datasets of fisheries over time.
Moreover, the combined effects derived from the longstanding interest in angling as well as the dependence of many populations on aquatic resources have made many fish stocks prised assets across nations.
As such, fish stocks are typically assessed annually or even seasonally by governments, private stakeholders, or international corporations. Thus, the long-term monitoring of catches and landings data makes fisheries an ideal system for applying computational models as the variations in data and consistent assessments will help develop accurate and detailed models.
The development of computational models in fisheries
The availability of long-term historical stock assessments is particularly valuable as characteristics such as catch per unit effort can be derived on a regional and even global basis and integrated into complex models.
This was achieved by an international team of researchers in a study published by the journal Nature in 2019. By combining data from animal movements and distribution with catch per unit effort, scientists were able to model the overlap of pelagic shark species and fishing efforts on a global scale. Changes over season, species, and fishing gear were then integrated to further understand the outcomes across models.
As a result, computational models produced an array of outcomes detailing the overlap between individual species over time and space that can be summarised in a key statistic – on average, nearly a quarter of pelagic sharks overlap monthly with longline fishers. The findings of this study will be critical in identifying vulnerable populations and regions of overexploitation, providing a wealth of knowledge for policymakers.
Moreover, such findings are being further refined as studies are now integrating even more complex data. For instance, the use of fine-scale distribution data can be superimposed on top of larger existing models. This will provide more local and precise information, ultimately improving the significance of findings.
The limitations and future of computational modeling
The primary limitation of computational models is the dependence on the availability of desired, accurate, and sufficient data to design the model reliably. Therefore, good quality data may produce good quality models, but poor-quality data will generate erroneous models and inaccurate findings.
The process of integrating several layers of information exemplified in the aforementioned study involved an unprecedented international collaboration (over 100 authors) gathering long-term datasets of both species and governmental fishing practices spanning several decades. This is often an impossible task and many models, therefore, rely on narrowing the spatial and temporal scales of integrated data.
However, developing computational models also requires key contextual considerations. This was illustrated in a study from 2004 led by Chris Thomas, which discussed the extinction risk of organisms in the face of global climate change.
Authors developed a series of models across taxa to understand the challenge of global warming and presented that up to 37% of species will face the risk of extinction by 2050. Taken at face value this figure is particularly concerning, but authors quickly realized several caveats within the data they had integrated.
Firstly, the models relied on the current distribution of organisms and superimposed the shifts in global temperature without considering the future distribution of the same organisms, ignoring any possible range shifts over time. Secondly, models also did not consider the physiological, behavioral, or developmental plasticity of organisms, which may compensate for temperature changes. Finally, thermal adaptation was also not considered as transgenerational changes were not integrated within the results.
The findings of the model are not incorrect, it simply lacks the depth and range of data necessary to develop a more accurate assessment. This illustrates the risk of developing and depending on computational models as the model output relies on the data inputted.
Ultimately, computational models offer considerable potential to understand environmental and anthropogenic stressors and their effects on systems such as fisheries. However, the potential of models relies on the quality and availability of relevant data. This is particularly important when considering large questions and datasets, as the questions asked must be refined as much as possible to obtain the correct answers.
Nevertheless, the application of computational models continues to improve as they are now frequently used to understanding environmental and socioeconomic changes. As such, despite the growing abundance and quality of data, the context and relevancy of data will remain essential.
Sources:
- Cao, J., Thorson, J. T., Punt, A. E., & Szuwalski, C. (2019). A novel spatiotemporal stock assessment framework to better address fine‐scale species distributions: Development and simulation testing. Fish and Fisheries, 21(2), 350–367. doi:10.1111/faf.12433
- Queiroz, N., Humphries, N. E., Couto, A., et al. (2019). Global spatial risk assessment of sharks under the footprint of fisheries. Nature, 572(7770), 461–466. doi:10.1038/s41586-019-1444-4
- Thiault, L., Mora, C., Cinner, J. E., Cheung, W. W. L., et al. (2019). Escaping the perfect storm of simultaneous climate change impacts on agriculture and marine fisheries. Science Advances, 5(11), eaaw9976. doi:10.1126/sciadv.aaw9976
- Thomas, C. D., Cameron, A., Green, R. E., et al. (2004). Extinction risk from climate change. Nature, 427(6970), 145–148. doi:10.1038/nature02121
Further Reading