Identifying genetic markers and patterns associated with certain traits make it possible to determine the probability that characteristics will be presented in an individual's phenotype. Utilizing this data to inform polygenic risk scores can reveal relative risks for "complex" or multifactorial diseases. However, the predictive capacity of these tools is currently very limited.
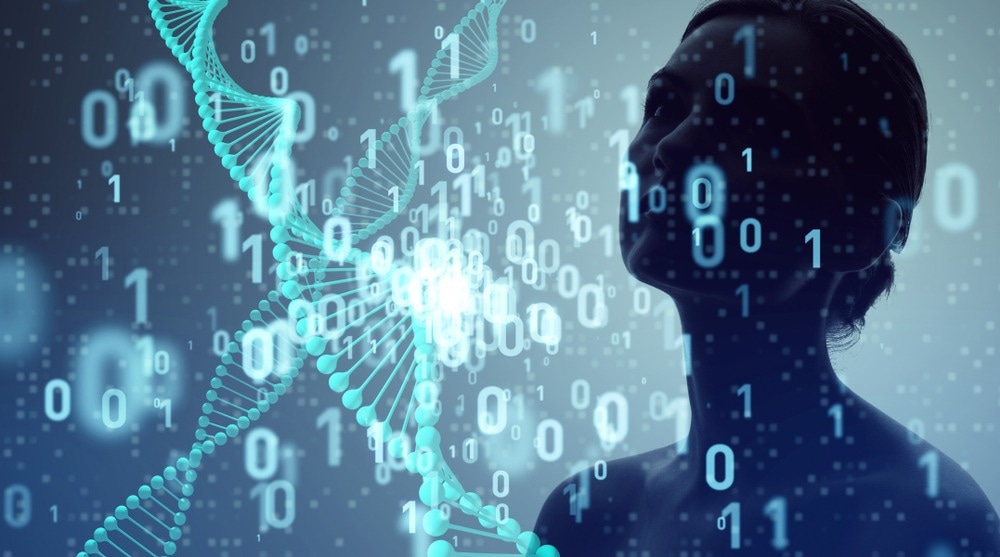
Image Credit: metamorworks/Shutterstock.com
Genome-wide association studies can analyze the genetic code and identify risk factors based on available databases. By utilizing next-generation sequencing technologies, genome-wide screening can rapidly determine an individual's genetic code and screen for known disease markers. Polygenic screening predicts characteristics and "complex traits" influenced by multiple genes.
As genetic sequencing becomes quicker and the tools required to become more accessible, their applications in clinical practice grow. But, the ability of these tools to determine an individual's risk for developing a particular disease is often limited by the genetic variants available in libraries. A complete and diverse reference genome is required to improve the analysis of human genetic variation.
NIH grants $38 million to improve diversity in genetic research
The lack of diversity in genomic databases often restricts the predictive accuracy of genetic screens. Disease-associated genetic variants, more commonly seen in underrepresented populations, can produce uncertain or false positive/negative results for individuals not of European ancestry. Consequently, clinical interventions, polygenic risk scores, and guidelines for risk reduction and the management of complex chronic diseases can be inaccurate, particularly for individuals from underrepresented groups.
In 2009, 96% of genome-wide association studies (GWAS) participants were of European descent. In 2017 this number had dropped by less than 10%, with individuals of non-European descent accounting for less than 13% of those participating in GWAS. As a response to this, the National Institutes of Health (NIH) funded the Polygenic RIsk MEthods in Diverse populations (PRIMED) Consortium; with the hope of improving predictions and outcomes for health and disease in diverse ancestry populations.
"One of our biggest concerns is that data used to calculate polygenic risk scores do not include sufficient numbers of individuals from diverse populations, falling short of effectively predicting disease risk in non-European populations," said Professor Teri Manolio (Director of the Division of Genomic Medicine at National Human Genome Research Institute).
Limitations of European bias in Genetic Research
The PRIMED Consortium aims to gather large datasets with genomic and health measures from populations of diverse ancestry. By expanding genomic libraries with annotated DNA sequences from underrepresented populations, the consortium hopes to improve the application of genetic screening and precision medicine, ultimately, with the aim of reducing the health care disparities experienced by minority groups. Improving the diversity of genomic libraries will also increase the quality of genomic research and the conclusion drawn for people of all backgrounds.
Expanding the diversity of genomic libraries will have implications beyond the healthcare of individuals of non-European ancestry. By improving genomic databases, association analyses and AI tools will be able to more accurately make predictions of an organism's phenotype based on its genotype. For individuals with rare variants, the increased diversity in data could help improve the power of tools to detect associations with clinically important phenotypes.
"Irrespective of what's driving [European bias in GWAS], the continued under-representation of populations of mixed ancestry or of people whose ancestry is not European is a problem." - Alice B. Popejoy (Ph.D. candidate at the University of Washington, USA), and Professor Stephanie M. Fullerton (Bioethics and Humanities, University of Washington, USA).
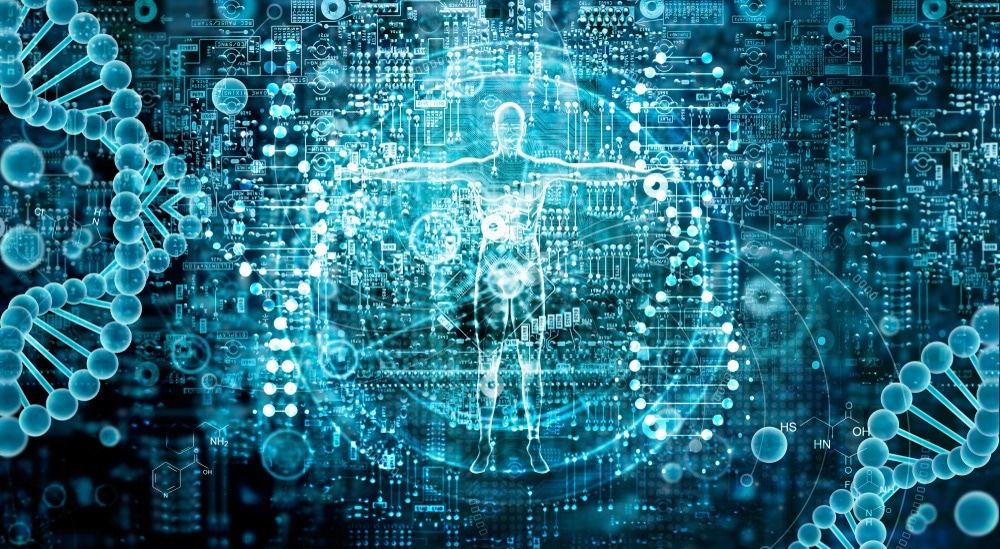
Image Credit: PopTika/Shutterstock.com
A tool of clinical medicine and public health
Large and diverse data sets have been demonstrated to improve the performance of models when applied to external data sets in different populations. Calculated as the weighted sum of risk alleles in a genome, polygenic risk scores use the strength of association between genomic markers and phenotypic effects to determine an individual's relative risk of disease. To improve the calculation of these scores, statistical models power computational tools to make predictions of health and disease, the accuracy of which is determined by training sets of GWAS data.
Polygenic screening data informs how predictive biomarkers for complex diseases can stratify patient populations according to risk. Using polygenic risk scores, clinicians can determine potential early preventative measures to offset the risk of disease and efficacious treatment options and health care plans. While the applications of polygenic risk scores in clinical practice are only just beginning to be explored, when discussing their potential to help doctors treat patients with iCommunity, Geneticists for the University of Queensland highlighted their ability to support precision medicine and individualized diagnosis.
I can see a day when someone arrives at a clinic presenting certain symptoms, and a PRS algorithm will be applied to their stored genetic data".
Professor Naomi Wray, Psychiatric and Quantitative Genetics, University of Queensland
Further Reading