Researchers from the chemistry and surgery departments at National Taiwan University in Taipei have conducted a study showing that combining an ambient ionization mass spectrometry (AIMS) approach with machine learning could speed up the diagnosis of breast cancer.
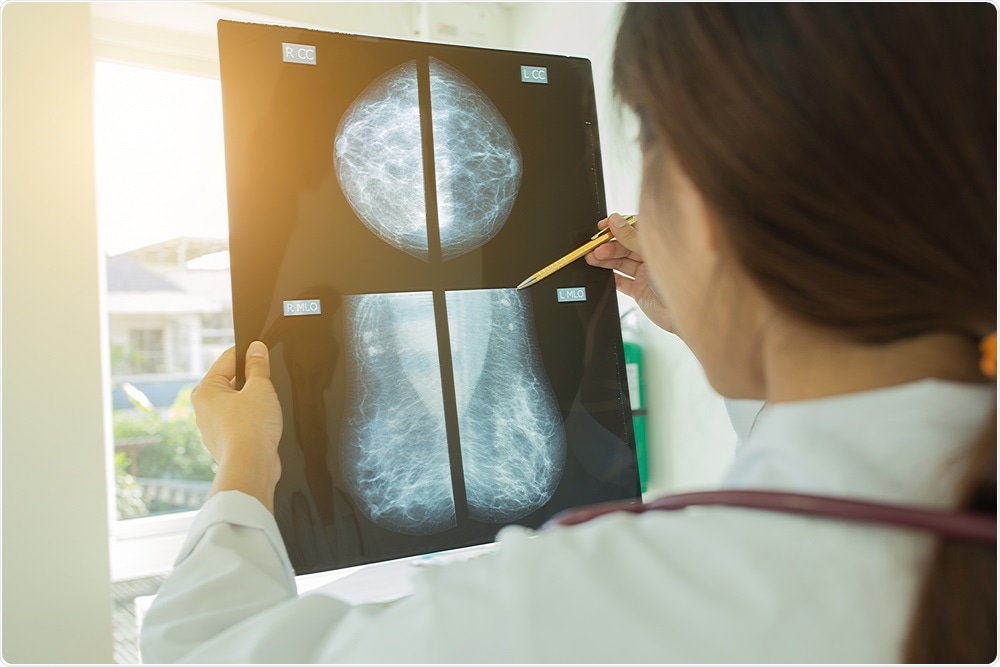
Image Credit: Chompoo Suriyo/Shutterstock.com
The researchers say the technique could provide a cost-effective and smart point-of-care testing (POCT) approach that could be used in the clinical setting.
The team’s paper, recently published in the journal Analytical Chemistry, describes an approach that combines a paper spray ionization mass spectrometry (PSI-MS) method with field asymmetric waveform ion mobility spectrometry (FAIMS) and a machine learning model called the least absolute shrinkage and selection operator (LASSO).
The current approach
Breast cancer is a leading cause of cancer worldwide. It affects millions of women every year and early detection is essential to improving prognosis and survival.
Currently, mammography and ultrasonography are used to detected suspected breast cancer in asymptomatic women, which, if identified, is followed up with a core needle biopsy to obtain a tissue sample that can be checked under the microscope.
This involves several time-consuming processes, including having to embed, section, fix and stain the sample using hematoxylin and eosin (H&E) and histopathological analysis can take as long as up to two weeks. Having to wait a long time for results can not only be very distressing for patients but is also associated with poorer outcomes.
Developing a rapid and sensitive diagnosis tool that could be performed within minutes is necessary and may eventually be translated to routine point-of-care testing (POCT) in clinics,”
Cheng-Chih Hsu and colleagues
Improving mass spectrometry approaches
Mass spectrometry measures the mass-to-charge ratio of molecular species in samples to create a chemical “fingerprint” of samples.
Over the last ten years, ambient ionization mass spectrometry (AIMS) techniques have been developed that require only minimal sample amount and preparation and provide real-time data on sample composition - features that hold great promise for cancer diagnostics.
While the use of AIMS could reduce the time patients must wait for results, the techniques are subject to the interference that results from high ion suppression. New strategies are therefore needed that can improve their performance.
One strategy is to couple ion mobility spectrometry (IMS) and field asymmetric waveform IMS (FAIMS) with the AIMS techniques, because this enables the separation of phase ions at atmospheric pressure and significantly improves the detection of metabolites and proteins.
What did this study involve?
For the current study, the team developed a PSI-FAIMS-MS platform for the interrogation of 202 breast core needle biopsy samples and combined it with a LASSO data classification model that was built using the MS results.
This platform can be readily implemented in regular clinical examinations in hospitals. After aspirating the tissue using a core needle biopsy, clinicians simply wipe the tissue into chromatography filter paper for subsequent chemical analysis by mass spectrometry technicians,”
Two tests were performed in which FAIMS conditions were optimized to enhance the signal intensities of biochemical components in benign and malignant samples (29 benign and 14 malignant in test 1; 117 benign and 42 malignant in test 2).
To validate the efficacy of the technique, the MS data were obtained under FAIMS-off and FAIMS-on conditions.
On comparing the MS data profiles for benign and malignant samples, the team found that potential cancer biomarkers could be identified. However, the large size and complexity of the datasets required informatics approaches and after testing different machine learning models, the researchers found the (LASSO) model was the best.
“Thus, in this study, we constructed a LASSO model for data classification and prediction using commercially available data science software,” they write.
The team says that compared with histopathological analysis with conventional H&E staining, which can take up to two weeks to generate a patient report, the PSI-FAIMS-MS platform provides an easy-to-operate alternative for diagnosing breast cancer that takes only minutes to produce results.
“Furthermore, the classification model built by the LASSO using the MS data performed with the accuracy of 87.5 % and could pinpoint important species related to breast cancer biochemistry,” they add.
High throughput, sensitive and accurate
The team says the proof-of-concept study shows that breast cancer can be readily diagnosed using the AIMS and machine learning combination: “The diagnostic metabolic and lipidomic features can be obtained in high throughput and sensitive manners with satisfactory predicting accuracy.”
The researchers suggest that in the future, generating a larger MS dataset from clinical samples could lead to the realization of a cost-effective solution with minimal expenditure for pathologists.
“Our results provide a direction towards fast, cheap, and smart POCT approaches that could be operated in clinics,” they conclude.
Journal reference:
Hsu C, et al. Predicting Breast Cancer by Paper Spray Ion Mobility Spectrometry Mass Spectrometry and Machine Learning. Analytical chemistry 2019 DOI: 10.1021/acs.analchem.9b03966