In this interview, we speak to Dr. Hari Shroff from the National Institute of Biomedical Imaging and Bioengineering about his latest research into confocal microscopy.
Please could you introduce yourself and tell us what provoked your latest research into artificial intelligence (AI) and confocal microscopy?
I am a tool developer who invents and then applies new forms of optical microscopy (particularly fluorescence microscopy) to problems in biology, with an emphasis on cell biology and neurodevelopment.
I’m constantly on the lookout for ways to improve the resolution, speed, signal-to-noise ratio (SNR), or gentleness of fluorescence microscopy – usually improving one of these attributes comes at the detriment of another. Artificial intelligence, and in particular deep learning, offer the potential to circumvent these tradeoffs by using prior information about the sample.
Given the recent renaissance in deep learning, we have begun to adopt these methods in our lab, and the integration with confocal microscopy is our latest effort to combine AI with microscopy.
Can you give us an overview of confocal microscopy, how it works, and some of its applications within the life sciences?
Confocal microscopy has been around for decades and is so useful that I suspect it will be around for many more. The idea is that a sharply focused illumination beam is scanned through the sample, eliciting fluorescence from each point (or line). This fluorescence is recorded on a detector, after being filtered through a pinhole (either a circular aperture or a slit).
The pinhole is ‘confocal’ with the illumination, meaning that it is in a conjugate optical plane. This confocality means that fluorescence originating at the focal plane is transmitted through the pinhole, but fluorescence emitted from outside the focal plane is greatly suppressed. This is the key advantage of confocal microscopy: the ability to interrogate densely labeled, thick samples where out-of-focus background and noise are suppressed, yielding clear images of 3D samples.
Within the life sciences, confocal microscopy is usually the first method of attack when imaging fluorescent samples thicker than a single cell.
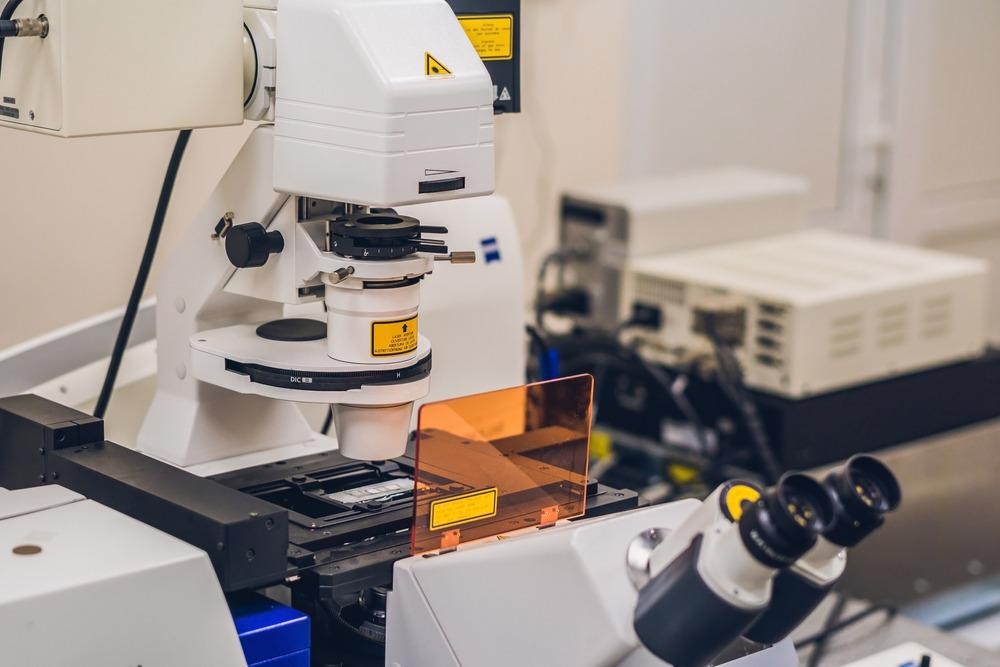
Image Credit: Elizaveta Galitckaia/Shutterstock.com
What advantages do confocal microscopes have over more traditional microscopy methods?
The main one is the ability to reject out-of-focus light, also called ‘optical sectioning’.
Despite their many advantages, they still have resolution issues. Can you describe some of the biggest challenges faced by confocal microscopes?
Besides spatial resolution being limited to the diffraction limit, another challenge is the 3D illumination of the sample. Even though the pinhole efficiently suppresses the out-of-focus fluorescence – it is still being generated throughout the entire sample. This leads to unwanted photobleaching (destruction of the fluorophore used to label the sample) and phototoxicity (limiting the length of experiments on live samples), forcing a compromise between illumination intensity/SNR and experiment duration.
Another challenge is that confocal microscopes build up the images point by point (or line by line), meaning a lower duty cycle (how much of the focal plane is generating useful signal) relative to more traditional approaches like wide-field microscopy.
And finally, the spatial resolution along the third dimension is lower than in the focal plane, because the objective lens used to image the sample collects higher resolution information laterally than axially.
In your latest research, you aimed to increase the performance level of confocal microscopy. How did you carry out this research?
On the instrumentation side, with our industry collaborators, we designed, built, tested, and deployed compact illuminators that delivered sharply focused line illumination to the sample and collected the fluorescence very efficiently, by synchronizing the motion of the illumination line with the detection readout of our camera. This allowed us to ‘add’ confocal capability easily to a microscope, and to collect more light than more classic confocal designs where there are more optical elements between the sample and the detector.
Perhaps more importantly, we positioned three of these illuminators around the sample, so that fluorescence could be collected along three directions – combining the information from these different views of the sample allowed us to improve resolution relative to classic confocal microscopes, which only collect the fluorescence from a single view. This ‘multiview’ capability also allowed us to compensate for scattering that reduced the fluorescence signal in thicker samples – by imaging from multiple sides we could compensate for the scattering that plagued any one view using data provided from the other two views.
We further improved spatial resolution by using approaches from structured illumination microscopy, a family of super-resolution techniques, to provide resolution ~2x better than the diffraction limit in each direction.
On the computational side, we developed deep learning pipelines that 1) denoised the data, allowing us to use lower illumination intensities; 2) used the data from a single viewing direction to predict the data from all three views, allowing us to improve speed and lessen phototoxicity/photobleaching; 3) obtained isotropic resolution enhancement from only one direction, also improving speed and lowering the dose.
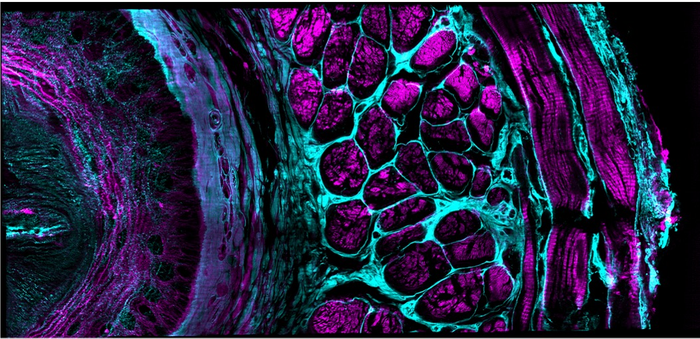
Image Credit: Yicong Wu and Xiaofei Han et al, Nature, 2021
What are the benefits of being able to use artificial intelligence algorithms within existing confocal microscopes?
I think that by properly using artificial intelligence, it is possible to considerably improve speed, reduce photobleaching/damage, and even improve spatial resolution in existing confocal microscopes. The benefit is that – given the large number of confocal microscopes in existence and plentiful GPU hardware for training neural networks – that these existing systems can deliver more ‘bang for the buck’ and that the end-user might not need to invest in a new microscope to achieve some benefit over their existing confocal system.
What applications does your new confocal platform have within life sciences? Do you believe that this new platform will help aid new discoveries in these areas?
Any time it is possible to ‘see more’ with a microscope, there is potential for biological discovery. To highlight a few examples from our paper, we were able to more accurately count nuclei and observe neuronal dynamics within living samples, quantify nuclei in fixed specimens, and image fine structure even deep into scattering samples. I suspect many other applications are possible, depending on the biology in question.
Do you believe that with increased resolution and performance within confocal microscopy, we will see more researchers beginning to use these microscopes in their research?
I think we will see more and more methods that integrate deep learning/artificial intelligence with confocal (and other optical) microscopes. Currently, these methods are mainly aimed at ‘image restoration’, i.e. given some performance tradeoff of a microscope, confocal or otherwise, can deep learning be used to ‘restore’ or ‘predict’ lost resolution, SNR, etc.?
Another exciting class of applications uses artificial intelligence to alter the experiment itself, in real-time or close to real-time – i.e., using prior information about the sample and tailoring the microscope’s parameters/operating procedure to maximize the chances of recording some biological event. This second class is just starting to take off, and I anticipate more growth in this area as well as the more classic image restoration applications.
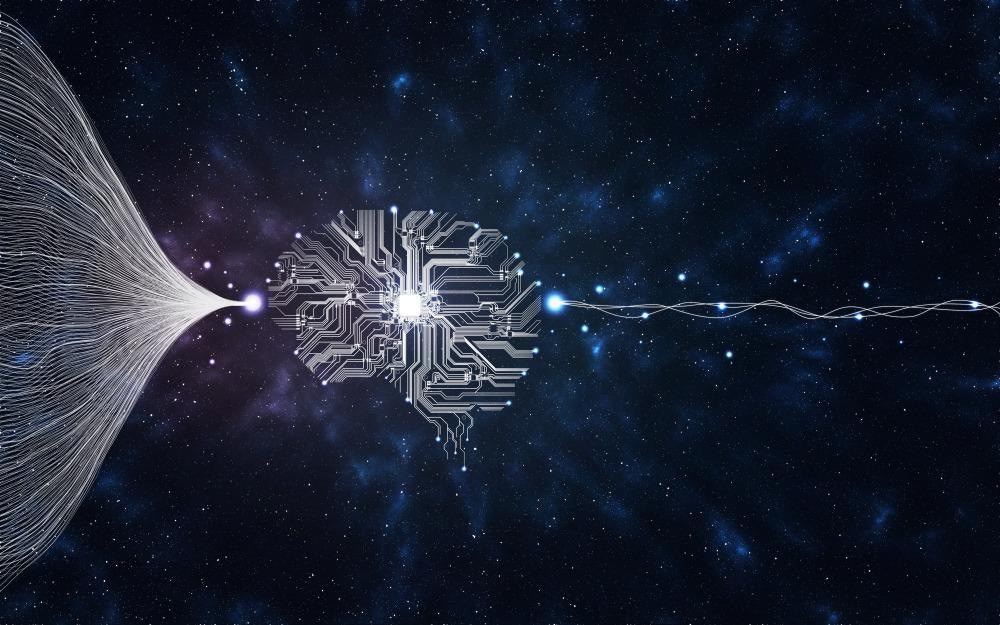
Image Credit: cono0430/Shutterstock.com
Artificial intelligence is becoming more prominent within the scientific community. What role does AI play within life sciences and how do you see this role changing over the next 20 years as technology develops?
I think AI has the potential to be transformative in life sciences generally, and in imaging in particular. This is because there really is a wealth of information that can be harnessed and put to use from previous experiments. I see two key challenges in this area.
First, we need better tools for quantifying (un)certainty with an AI prediction. Although it is often said that ‘seeing is believing’, I think a better question might be, ‘how can we believe what we see’? The results of these deep learning networks are only ‘predictions’ (albeit often very good ones). A key challenge to overcome is in convincing biologists or end-users when these predictions succeed (or fail) at delivering good predictions.
Second, I think the end-game for the image restoration approaches is to bypass the image itself because at the end of the day the biologist doesn’t actually care about the image – they care about some feature of the image, or hypothesis that can be drawn about their experiment from the image. Can we build approaches that bypass the image completely, producing the ‘answer’ to a hypothesis with useful bounds on the error on the answer? Might sound like science fiction now, but perhaps not in twenty years…
What are the next steps for you and your microscopy research?
We have some ideas for further improving the performance of our multiview confocal platform that we are currently pursuing, and I’m also excited to apply the same general approach to other microscopy platforms (i.e., non-confocal).
We’re also working on better ways of doing adaptive optics, a class of methods that correct for distortions in the fluorescence due to sample-induced optical aberrations. And finally, we have a longstanding interest in applying all these methods to studying brain development in worm embryos.
Where can readers find more information?
The paper: Multiview confocal super-resolution microscopy | Nature
Some nice writeups about the work from NIBIB, my home institution: Catching all the action from twitch to hatch (nih.gov)
And the MBL at Woods Hole: Enhancing the workhorse: Artificial intelligence | EurekAlert!
To read some early applications of this method, click here:
About Dr. Hari Shroff
Dr. Hari Shroff received a B.S.E. in bioengineering from the University of Washington in 2001, and under the supervision of Dr. Jan Liphardt, completed his Ph.D. in biophysics at the University of California at Berkeley in 2006. He spent the next three years performing postdoctoral research under the mentorship of Eric Betzig at the Howard Hughes Medical Institute's Janelia Farm Research Campus where his research focused on the development of photoactivated localization microscopy (PALM), an optical superresolution technique.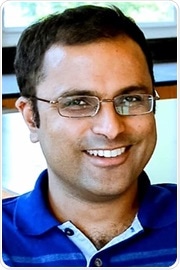
Dr. Shroff is now chief of NIBIB's Laboratory of High Resolution Optical Imaging, where he and his staff are developing new imaging tools for application in biological and clinical research.