Antibiotic resistance is a growing problem in treating bacterial infections. Specific bacteria in a colony can develop resistance to a certain antibiotic being used to treat it, and the resistance can then spread quickly through the bacterial population.
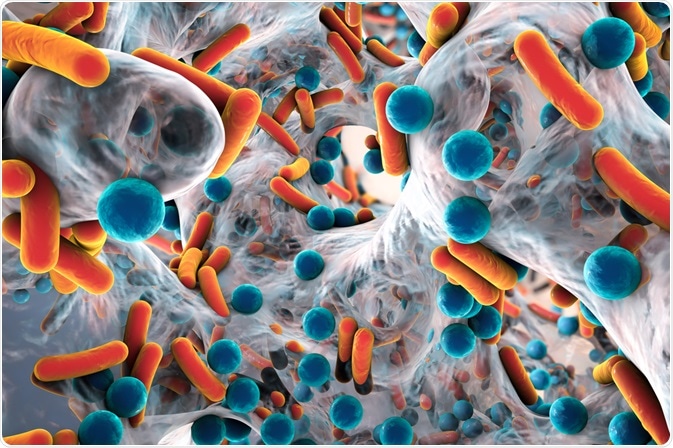
Image Credit: Kateryna Kon/Shutterstock.com
Resistance often means second-line antibiotics must be used to treat a patient. Such antibiotics are often less effective and can cause significant side effects. This can lead to longer treatments, making it more difficult for the patient to recover.
Where bacteria develop resistance to two or more antibiotics, it can make it very difficult to treat infections.
For example, in tuberculosis, resistance to first-line drugs (particularly rifampicin and isoniazid) has given rise to multiple drug-resistant tuberculosis which can increase treatment time from 9 to 18 months, while second-line drugs can cause harmful side effects such as nausea, diarrhea, and cardiac problems.
Overuse of antibiotics in animal agriculture, and misuse in general practice, has led to rising rates of antibiotic resistance. Therefore, new antibiotics are desperately needed. Traditional methods of development include experimental chemical synthesis and isolation of natural antibiotics.
However, over the past few decades, these methods have resulted in very few new antibiotics. There is often a high degree of error and it can be a very costly approach for pharmaceutical companies.
Artificial intelligence in antibiotic discovery
The purpose of using artificial intelligence is to enable predictions of new antibiotics that are structurally distinct from current antibiotics and that are also effective across a broad spectrum of bacteria. An antibiotic candidate would then be synthesized from a model and tested for antibiotic activity.
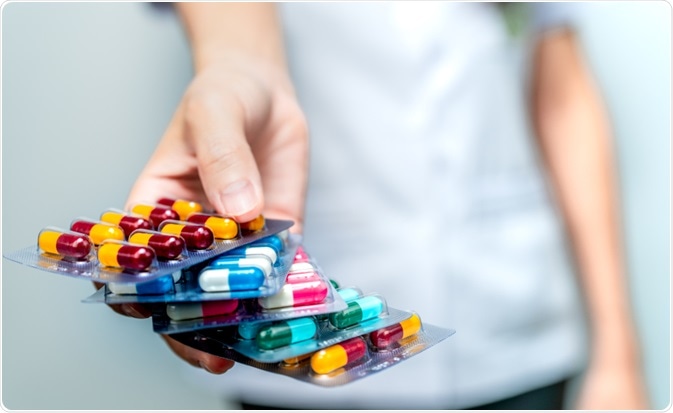
Image Credit: Fahroni/Shutterstock.com
Artificial neural networks have previously been used in this process. They are computing systems loosely based on biological neural networks that allow the system to ‘learn’. This permits a chemically accurate molecular structure to be predicted, which was not possible beforehand.
An artificial neural network can be trained to predict growth inhibition of a specific bacterial species based on a relatively small collection of molecules (2-3,000). This understanding can then be applied to much larger chemical libraries (>100 million molecules) to identify potential antibiotic candidates.
This reduces a lot of the trial and error involved in antibiotics development. A computing system such as this could predict antibiotic chemical structures with a high degree of accuracy, reducing resources spent on developing compounds with little antibiotic activity.
Discovery of halicin
A group of researchers at the Massachusetts Institute of Technology (MIT) have used this approach to identify the compound halicin, a c-Jun N-terminal kinase inhibitor. Halicin has subsequently shown bactericidal effects against drug-resistant strains of tuberculosis and carbapenem-resistant Enterobacteriaceae (CRE).
The ability to act against drug-resistant bacteria is partly due to an unusual mechanism of activity. Halicin alters the pH gradient across the bacterial cell membrane and therefore disrupts a variety of activities (including the production of ATP) that are essential for bacteria survival.
Halicin was subsequently tested in vivo in a mouse model infected with a strain of Acinetobacter baumannii resistant to all current antibiotics. Within 24 hours of application, the infection was completely cleared.
Additionally, resistance was shown to not develop in treated bacteria. Over a 30-day treatment period, Escherichia coli didn’t develop any resistance to halicin. Conversely, a control group treated with ciprofloxacin developed resistance within 1-3 days.
Halicin is still in the very early stages of development, with its safety and efficacy in humans yet to be determined. However, these initial results are very promising, particularly in its demonstrated bactericidal ability against multiple drug-resistant strains of bacteria.
The discovery of such a potentially powerful antibiotic early on in the use of artificial intelligence indicates the possibility for many other broad-spectrum antibiotics to be identified, which could be useful against a broad spectrum of bacteria.
Application of the technology to different, larger databases has helped to identify other potential antibiotic candidates. The database ZINC5 (including around 1.5 billion chemical compounds) was screened, finding 23 potential antibiotics that are structurally distinct from existing ones.
Conclusion
While the discovery of new antibiotics using artificial intelligence is exciting, there are many improvements within the technology and its use that could lead to further discoveries.
Computing is an evolving discipline and its application in chemical research is relatively new. Different approaches to the use of artificial intelligence in antibiotic discovery may yield even more exciting results.
It is also worth stating that artificial intelligence is also useful in other aspects of preventing further increases in antibiotic resistance. Its role in understanding the epidemiology of resistance is key to preventing further exacerbation of the issue.
Developing new antibiotics will be crucial to preventing deaths from resistant bacteria, and artificial intelligence is an excellent tool for this. Nevertheless, this approach should be combined with preventative strategies against the spread of resistance, as even with this technology there are only a finite number of different antibiotic compounds.
References
- news.mit.edu (2020) Artificial intelligence yields new antibiotic | MIT News. Available at: https://news.mit.edu/2020/artificial-intelligence-identifies-new-antibiotic-0220 (Accessed: 18 March 2020).
- Rodríguez-González, A., Zanin, M. and Menasalvas-Ruiz, E. (2019) ‘Public Health and Epidemiology Informatics: Can Artificial Intelligence Help Future Global Challenges? An Overview of Antimicrobial Resistance and Impact of Climate Change in Disease Epidemiology’, Yearbook of medical informatics. NLM (Medline), 28(1), pp. 224–231. doi: 10.1055/s-0039-1677910.
- Stokes, J. M. et al. (2020) ‘A Deep Learning Approach to Antibiotic Discovery’, Cell. Cell Press, 180(4), pp. 688-702.e13. doi: 10.1016/j.cell.2020.01.021.
Further Reading