Polygenic risk scores (PRS) are promising tools for forecasting disease risk, but current versions have bias built-in, which can reduce their accuracy in some populations and lead to health disparities.
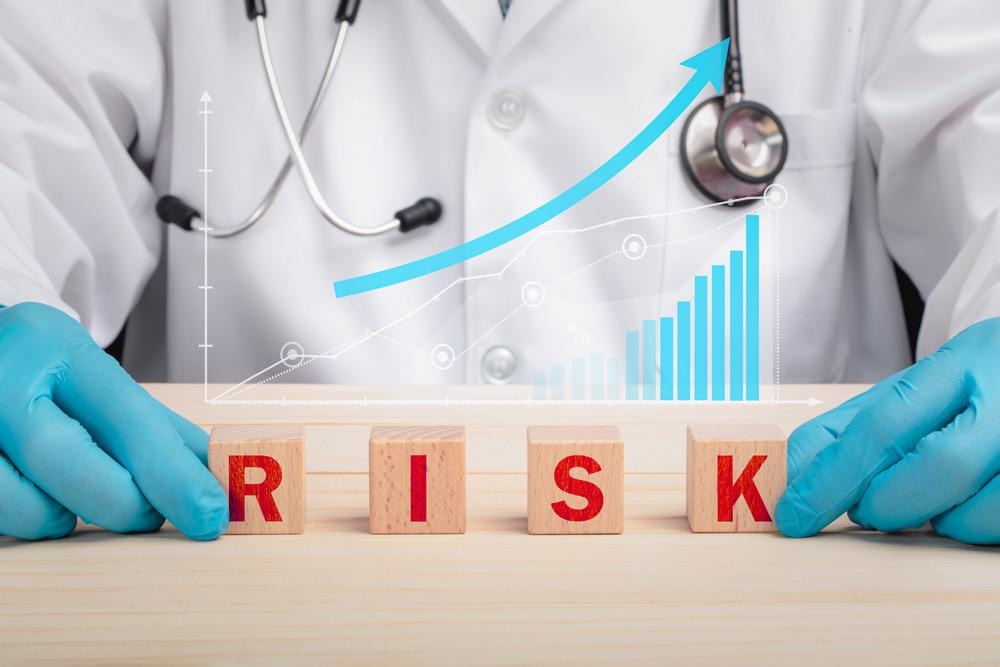
Image Credit: Tomatheart/Shutterstock.com
However, a group of researchers from Massachusetts General Hospital (MGH), the Broad Institute of MIT and Harvard, and Shanghai Jiao from Tong University in Shanghai, China, have developed a novel way of producing PRS that more correctly predicts disease risk across populations. The study was reported in the journal Nature Genetics.
Changes in the DNA sequence of a gene can result in a genetic variation that raises the risk of disease. Some genetic variants, like the BRCA1 mutation and breast cancer, have been related to specific diseases.
However, most common human diseases—such as type 2 diabetes, high blood pressure, and depression, for example—are influenced not by single genes, but by hundreds or thousands of genetic variants across the genome. Each variant contributes a small effect.”
Tian Ge, PhD, Study Co-Senior Author, Center for Genomic Medicine, Massachusetts General Hospital
Ge is a biostatistician and applied mathematician at MGH’s Psychiatric and Neurodevelopmental Genetics Unit.
PRS combine the impacts of gene variants across the genome and has shown promise in the future for predicting disease risk in individual patients. Clinicians would be able to propose preventative measures and constantly monitor patients for early detection and intervention.
A PRS, on the other hand, must be “trained” to forecast disease risk using data from studies that collect genomic data from large groups of people. While many disease-causing variations are similar, according to Ge, the genetic foundation of disease differs significantly amongst people of various ancestries.
A frequent genetic variant linked to a certain disease in one community may have a lower frequency or perhaps be absent in another. According to Ge, when a disease-linked genetic variant is common among populations, its impact size, or how much it raises the risk, might differ from one ancestral group to the next. When PRS are trained on data from one population, their performance is generally attenuated or diminished when applied to other populations.
“A major problem with existing methods for PRS calculation is that, to date, most of the genomic studies used data collected from individuals of European ancestry,” adds Ge.
Ge claims that this leads to a Eurocentric bias in present PRS, resulting in much less accurate predictions and the likelihood of over- or underestimating disease risk among non-European populations.
Fortunately, researchers are working harder to obtain genetic data from underrepresented groups. Using these resources, Ge and their research colleagues developed PRS-CSx, a new tool that can combine data from multiple populations while accounting for genetic similarities and differences.
The researchers employed computational approaches to optimize the utility of non-European data and increase prediction accuracy in ancestrally diverse individuals, even though there is still much more genetic data on people of European ancestry.
The researchers analyzed genetic data from people from various populations to predict a variety of physical characteristics (such as height, BMI, and blood pressure), blood biomarkers (like glucose and cholesterol), and the risk of schizophrenia.
Researchers next measured PRS-CSx’s prediction accuracy by comparing predicted trait or disease risk to actual measurements or reported disease status. In non-European populations, the results showed that PRS-CSx is much more accurate than existing PRS instruments.
The goal of our work was to narrow the gap between the prediction accuracy in underrepresented populations relative to European individuals, and narrow the gap in health disparities when implementing PRS in clinical settings.”
Tian Ge, PhD, Study Co-Senior Author, Center for Genomic Medicine, Massachusetts General Hospital
Ge says the new tool will continue to be enhanced in the hopes that physicians would be able to utilize it to influence treatment decisions and make patient care recommendations one day.
According to the study’s principal author, Yunfeng Ruan, PhD, a postdoctoral research associate at the Broad Institute of MIT and Harvard, PRS-CSx might play a role in fundamental research. It might be used to investigate gene-environment interactions, such as how the influence of genetic risk in global populations varies depending on the degree of environmental risk factors.
Even with PRS-CSx, there is still a significant difference in prediction accuracy between European and non-European populations. To increase PRS prediction accuracy in different populations, increasing sample diversity across worldwide populations is critical.
The expansion of non-European genomic resources, coupled with advanced analytic methods like PRS-CSx, will accelerate the equitable deployment of PRS in clinical settings.”
Hailiang Huang, PhD, Study Co-Senior Author, Statistical Geneticist in the Analytic and Translational Genetics Unit, Massachusetts General Hospital
Huang is also a member of the Stanley Center for Psychiatric Research at the Broad Institute.
Ge is also a psychiatry assistant professor at Harvard Medical School (HMS). Huang is a medical assistant professor at HMS.
Source:
Journal reference:
Ruan, Y., et al. (2022) Improving polygenic prediction in ancestrally diverse populations. Nature Genetics. doi.org/10.1038/s41588-022-01054-7.