In this interview, we speak to SLAS 2023 keynote speaker Joshua Smith from IBM Research about the importance of artificial intelligence (AI) in accelerating the discovery of new therapeutics and biomarkers.
Please can you introduce yourself and tell us what inspired your career in the life sciences?
My name is Joshua Smith, and I'm currently the Global Lead for Industry Partnerships for Accelerated Discovery at IBM Research. My background and training are actually in electrical engineering—more hardware related. As far as what inspired my career trajectory toward life sciences, that's an interesting question. Like so many, I think my career path is the result of many influences along the way. But I suppose, at the core, what inspired my decisions along that journey was an underlying desire to make a difference—to have some lasting impact.
My PhD work was more narrowly focused on low-dimensional nanoelectronics. That brought a natural gravity toward IBM Research, where I continued this path when I graduated. But I quickly found that a knowledge of semiconductors was in demand there, at that time, for creating microfluidic devices useful in the life sciences space. What's more impactful than helping to make devices that can potentially save lives, right?
Eventually, I became interested in learning more about the AI capabilities being developed in this space, so I took a position as the Technical Assistant to the Director of Healthcare and Lifesciences. When I completed my time in that role, I joined a then-budding Accelerated Discovery team tasked with delivering these AI capabilities at scale.
You are the Global Lead for Industry Partnerships Accelerated Discovery Strategic Partnerships and Operation Research at IBM TJ Watson Research Center. Tell us more about your role and the work you are currently involved in?
So, think of me as a matchmaker. We are building capabilities designed to enable users—scientists, and developers, placing at their fingertips the latest and most advanced methods to accelerate science. This includes game-changing technologies like AI, hybrid cloud, and quantum computing, enabling fundamental AI-accelerating technologies. This includes knowledge integration at scale, AI-enabled simulation, generative AI hypothesis generation, and automated synthesis.
Our partners, which include a host of academic, industry, startups, and government entities, are generally the users and validators of these technologies within their workflows and sometimes the creators of their own AI models and technology. They bring domain expertise.
A primary job role for me is to meet with potential and existing partners to find synergies, with IBM Research as the provider of technologies from which partners can derive added value.
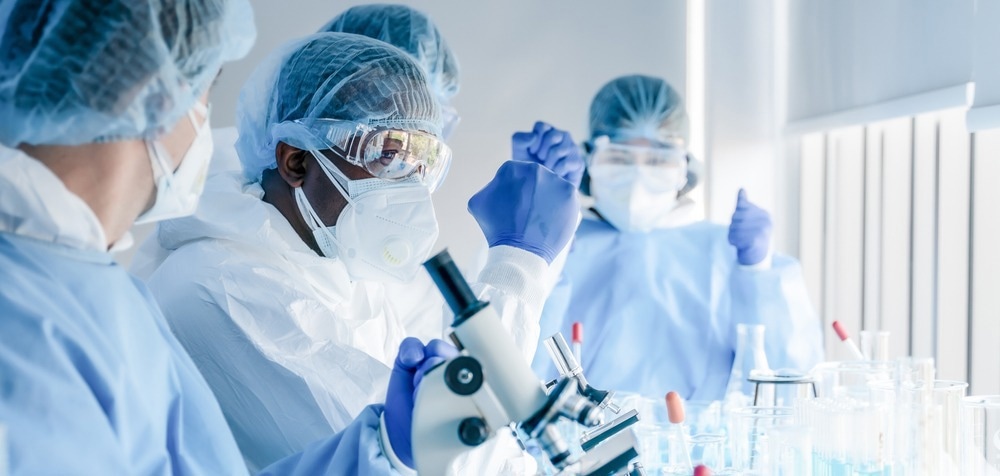
Image Credit: Mongkolchon Akesin/Shutterstock.com
The life sciences has evolved significantly in recent years, largely due to the acceleration of collaborative research and partnerships. How important do you believe partnerships are to life sciences research, and why is it important to also foster relationships between academia and industry?
That's a great question. Yeah, these relationships and, even more broadly speaking, open communities of discovery have played a critical role in this evolution. Ultimately, it's technology that drives acceleration. And partners help harden and validate new tech in real-world settings. Their insight is invaluable, and as part of this, but again on a grander scale, I believe that open-source communities have and will continue to be crucial in this regard.
These relationships must be fostered because ecosystems are where discovery is made, which is why IBM is such a strong advocate for open science communities. In fact, at SciPy 2022, we announced together with NumFOCUS, an Open-Source Science (OSSci) Initiative that convenes developers, and the best of the open-source community, together with the methods and rigor of science.
There is a rapidly growing list of partners who have embraced this vision and joined the community. Why did we do this? Well, science has always driven technology, yet, over the past couple of decades, technology has advanced so quickly, and science has become so complex, distributed, and challenging that these worlds are now out of sync. So, this is really an aspiration to convene these two great community efforts of the modern era by bringing together scientists and technology developers to drive a new open era of progress.
The life sciences has also seen an explosion in available data due to technological advancements. Why has there been such an increase in health-related data, and how can we use this data to accelerate therapeutic discoveries?
The rapid increase in the amount of health-related data being generated and collected is, in large part, due to an increasing number of data sources and data volumes that are coming from consumer devices, including wearables, mobile apps, and connected medical devices, as well as from things like EHR systems, genomic research initiatives, etc.
And there are a number of generalizable AI capabilities that can take advantage of this vast ocean of data to enable users to go from idea to impact faster. These include capabilities like knowledge integration that allows users to create knowledge graphs from existing scientific literature and query against that vast body of information, AI-enriched simulation to fill gaps in existing data, generative AI for hypothesis generation, and automated synthesis and testing. Together these AI capabilities can accelerate entire workflows.
Among these, one of the most exciting macro trends that we are seeing today is in the area of large language models—a type of foundation model trained on enormous quantities of unlabeled data, generally in a self-supervised way. By now, everyone is familiar with ChatGPT and has seen what generative AI is capable of using a foundation model approach. Beyond language, foundation models can also be applied to the language of chemistry, with enormous potential for materials discovery research, including drug discovery. For example, lead generation and optimization. I think we will see a lot more here in the future.
Despite this increase in available data, unlocking its true research potential has proven challenging. Why is this, and how can we use innovative tools such as AI and cloud computing to help gain deeper insights?
To gain deeper insights, I think that there are really three game-changing technologies that will help get us there, which I mentioned previously: cloud computing, AI, and quantum.
Cloud computing has democratized the affordability and access to computing.
AI foundation model approaches provide representations that become meaningful for many downstream applications that you can then create generative AI models from in a much more compressed time frame, as I've described. They offer generalizable and adaptable learned representations. Before about 2017, AI was really bespoke—task-specific and required a lot of training, labeling, and human involvement. The advent of deep learning around 2010 helped, but what we see now is the next level.
Quantum is poised to tackle problems that are simply intractable for classical computers, including key problems related to simulating nature—problems of chemistry, material science, and physics. The challenge is that sometimes knowledge is inaccurate because conducting certain types of experiments in the real world or simulating their outcomes is impossible due to their complexity. Quantum will allow us to augment knowledge gaps for these very complex problems outside the scope of what is currently possible. We are seeing quantum now reaching sufficient maturity to have an impact here, and we will start seeing quantum advantage for a growing number of use cases in the next few years.
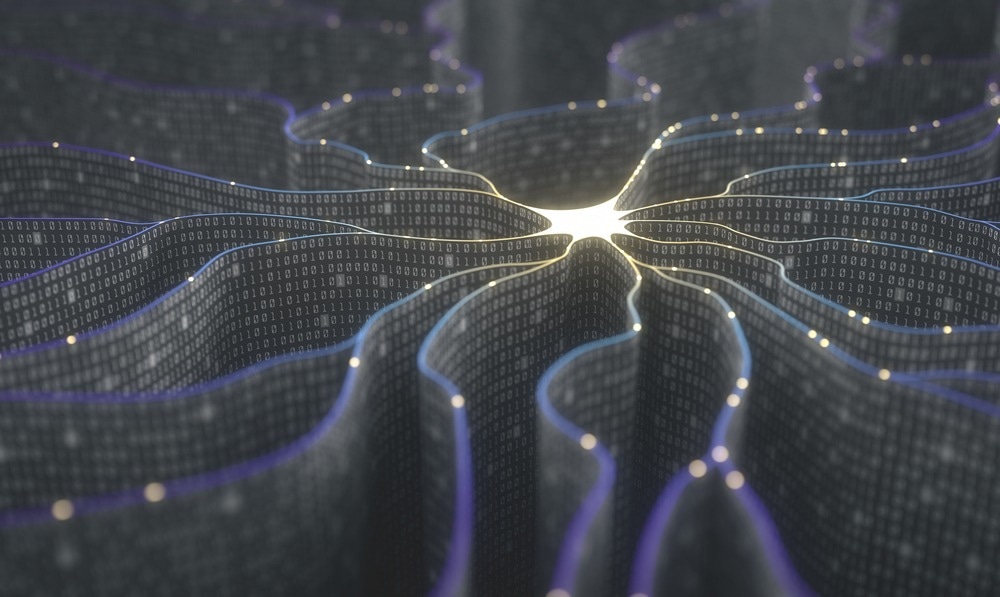
Image Credit: ktsdesign/Shutterstock.com
You are a keynote speaker at SLAS 2023 and giving a talk titled 'Does AI Accelerate the Discovery of Therapeutics and Biomarkers?'. Please can you give an overview of what you will be discussing in this talk and what listeners can expect to learn?
My talk discusses cloud, AI, and quantum and how these come together. Interestingly, each of these is hitting inflection points nearly simultaneously. The combination of these is greater than the sum of the parts and will completely transform IT to the point where we can accelerate workflows by a significant amount, even ten times.
The talk will also discuss everything from knowledge ingestion and AI-enabled data augmentation to generative AI and autonomous lab testing. These will significantly reduce cost and time. For example, going from a solution that might take ten years to just one year—is a critical necessity in a pandemic.
My desire would be for listeners to walk away with a more holistic view of how these technologies can converge—all to reduce time-to-result and cost for major, complex societal problems like drug discovery.
Joshua T. Smith - SLAS 2023
Joshua T. Smith - SLAS 2023 from AZoNetwork on Vimeo.
Are you hopeful that with the continued adoption of AI technologies, we will see new breakthroughs being made within the field of drug discovery? What would this mean for both research and patients?
Absolutely. Yeah, I really think it's a question of when, not if, for more integrated adoption of AI in drug discovery and development. It will be breakthroughs validated by earlier adopters that will convince other researchers. Earlier adopters are always the ones with the most urgency—those who recognize a huge pain point in their workflows and are hungry for an alternative. The ability to create AI models like generative to simplify a scientific task at scale for a reasonable investment and much faster is what is going to make a difference. Demonstrations of this will make converts in the larger community.
What would this mean for research and patients? For researchers, it means faster, cheaper solutions in a fraction of the time. For patients, it may mean more targeted, personalized medicine at a lower cost.
With partnerships being so fundamental to accelerating scientific research, how important are in-person conferences such as SLAS in nurturing relationships between sectors?
They are critical. I love technology, so don't get me wrong—it's fantastic that we found new ways of working as a result of adaptations to COVID. But being able to meet in person facilitates more intimate and open conversation. I think this is key for idea generation and collaboration. It brings some of the creativity back into what we are doing, and this bolsters ecosystems and partnerships alike.
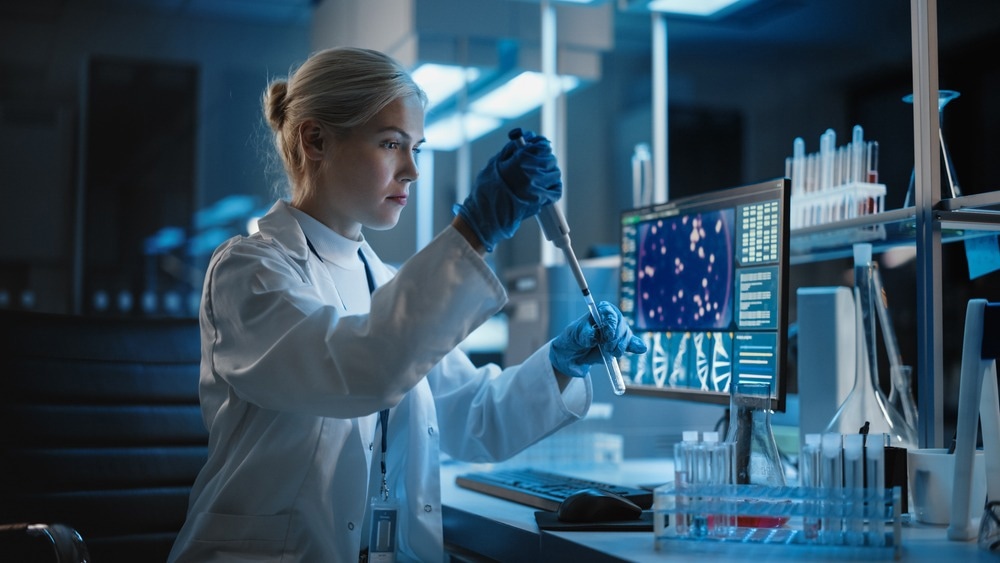
Image Credit: Gorodenkoff/Shutterstock.com
What is next for you and your work at IBM? Are you involved in any exciting upcoming projects?
We are working on many exciting projects linked to a 10-year partnership with Cleveland Clinic. Here, both sides have come together, with IBM bringing technology and Cleveland Clinic being the validator and bringing significant expertise on their end. This work mostly focuses on drug discovery and digital health applications, which we are very excited about. There is much more to come here in the coming months and years.
About Joshua Smith
Joshua Smith received his Ph.D. in Electrical Engineering from Purdue University in 2011 on a National Science Foundation Graduate Research Fellowship Award, joining the IBM T. J. Watson Research Center as a Research Staff Member. With a background and training in low-dimensional nanoelectronics, Dr. Smith developed a growing interest in biomedical engineering and biotechnology, and in 2013 he helped establish the Translational Systems Biology and Nanobiotechnology Group at IBM Research and later managed the Molecular Health Solutions Group, overseeing R & D efforts for microfluidic devices aimed at separation and detection of single molecules for advanced biomedical diagnostics and preparative technology solutions. After serving as the technical assistant to the Vice President of Healthcare and Life Sciences at IBM Research from Nov 2020 to Jan 2022, he joined the Accelerated Discovery team focused on holistic acceleration of scientific discovery workflows for healthcare and materials research. 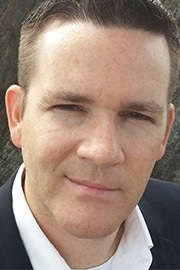
Dr. Smith has held an Adjunct Assistant Professor position at Columbia University in the Department of Electrical Engineering and is an IBM Master Inventor with more than 80 filed patent applications and over 50 granted patents. He has co-authored 22 peer-reviewed journal articles, and his research has been highlighted by Forbes, CNN Money, IEEE Spectrum, and Pharma Technology Focus among other media outlets as well as on-stage at TED.