DeMAG is a new method that was developed by Max Planck and Harvard’s research team. It is a new technique that has been shared as an open-source web server (demag.org) to assist interpret mutations in disease genes and enhance clinical decision-making.
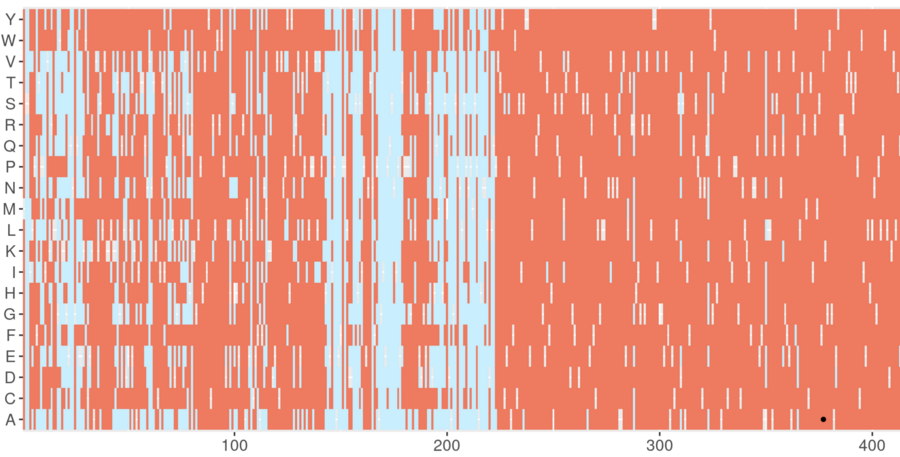
Screenshot from the DeMAG webserver. DeMAG predicts benign mutations in light blue and pathogenic ones in coral. Effects of all possible amino acid mutations are illustrated with the 20 alphabet letters on the y-axis at each position in a given protein, which is shown on the x-axis from position 1 to 425. Image Credit: Agnes Toth-Petroczy, Nature Communications, 2023/MPI-CBG.
Despite the high usage of genomic sequencing in clinical practice, rendering rare genetic mutations, even among well-studied disease genes, is a hard thing. The present predictive models are beneficial for interpreting such mutations, but they are prone to misclassify those that do not cause diseases, adding up to false positives.
Scientists from the Max Planck Institute of Molecular Cell Biology and Genetics (MPI-CBG) in Dresden, the Center for Systems Biology Dresden (CSBD) in Germany, and the Harvard Medical School in Boston, USA, have come up with a tool known as Deciphering Mutations in Actionable Genes (DeMAG) reported in the Nature Communications journal.
DeMAG is known as an open-source web server (demag.org) that provides an interpretation of the effects of all possible single amino acid mutations that could happen in 316 clinically appropriate genes that cause diseases for which preventive treatments and diagnostics are available from early on.
DeMAG offers medical professionals a tool that enables them to more precisely evaluate the effect of mutations in those genes by decreasing the false positive rate, thereby implying that less benign mutations are forecasted as pathogenic. Consequently, the tool has the potential to assist clinical decision-making.
In the past few years, genomic sequencing has turned out to be affordable and highly advanced. From one point of view, this enables clinicians to highly utilize sequencing for diagnostic purposes while also enabling researchers to explore more research hypotheses.
As the first option, several detected mutations do not contain a clear clinical interpretation. The doubt over whether a mutation causes disease could be stressful for patients and result in psychological burden, morbidity, and health-care expenses linked to under- and overdiagnosis.
While present tools are already utilized to forecast the functional impact of such variants, their performance has been biased as a result of the limited clinical data that makes differentiating between pathogenic (disease-causing) and benign (neutral) variants within a provided gene and frequently results in misclassifying mutations that do not lead to disease as pathogenic. Fulfilling such difficulties is vital for coming up with a trustworthy predictor for clinical applications.
The research group of Agnes Toth-Petroczy at the MPI-CBG and the CSBD worked with Christopher Cassa, Assistant Professor of Medicine at the Brigham and Women’s Hospital Division of Genetics at the Harvard Medical School, and Ivan Adzhubei, research associate at the Department of Biomedical Informatics at Harvard Medical School, to come up with a statistical model and web server DeMAG that reaches high precision in the interpretation of genetic mutations in disease genes.
For this to be done, the scientists carefully chose known pathogenic and benign mutations for the model to be trained.
We used clinical and various population databases. We selected only mutations whose clinical interpretation is agreed upon among multiple submitters such as medical doctors and genetics laboratories. And we also included data from ancestries that are underrepresented in the current population databases, such as Korean or Japanese, to make it even more representative and accurate.”
Federica Luppino, Study First Author and PhD Student, Max Planck Institute of Molecular Cell Biology and Genetics
DeMAG includes a novel feature called the “partners score”, that determines clusters of amino acids present in a protein that share the same clinical effect. With the partners score, DeMAG takes benefit of the amino acid relationships depending on evolutionary information from the genomes of several organisms and the recent AI (Artificial Intelligence) revolution of forecasting the 3D shapes of proteins with the help of the AlphaFold algorithm that has been developed by Google DeepMind.
We provide a basic framework for integrating clinical and protein data to aid assessing the impact of mutations. We hope that our tool and web server will ease variants effect assessment and clinical decision-making. Furthermore, the newly developed features can be applied to other genes and organisms beyond humans.”
Agnes Toth-Petroczy, Max Planck Institute of Molecular Cell Biology and Genetics
Source:
Journal reference:
Luppino, F., et al. (2023). DeMAG predicts the effects of variants in clinically actionable genes by integrating structural and evolutionary epistatic features. Nature Communications. doi.org/10.1038/s41467-023-37661-z.