The role of in silico strategies in drug discovery and design is expanding. Computational methods have applications at multiple levels of drug design and offer respite for the dependence on animal models in pharmacological research. Aiding the rational design of novel and safe drug candidates, as well as supporting the discovery of new applications for existing drugs, in silico strategies offer a cost-effective and rapid method of validation.
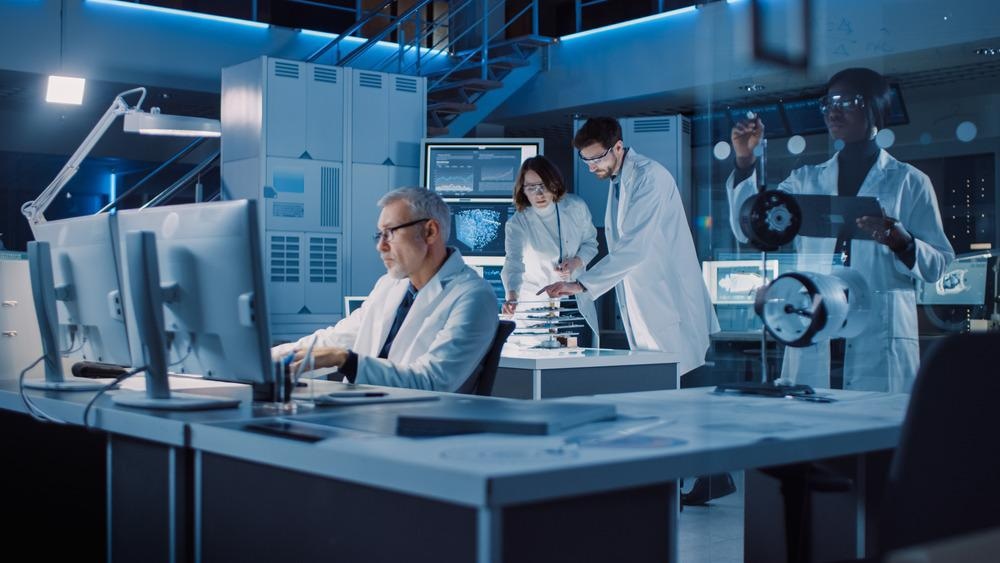
Image Credit: Gorodenkoff/Shutterstock.com
With two primary paths to drug discovery, compounds with physiological effects are identified by either: the desirable influence the molecule exerts on the human body; or, by the molecule's ability to modulate the activity of a target. Compounds with desirable physiological effects are often the subject of ligand-based drug design.
Ligand-based drug design (LBDD)
Structural information about compounds of interest, obtained from X-ray diffraction, nuclear magnetic resonance (NMR), or molecular simulation can inform computational models. These models define the conformation and interactions required of a molecule to bind and modulate the activity of its target. Quantitative structure-activity relationships (QSAR) can be utilized in LBDD to establish a mathematical relationship between chemical structure and its biological activity on a target.
QSAR models are one of the most advanced AI techniques, allowing for elucidation of potential mechanisms of action. Algorithm-based tools can also be used to predict the chemical, biological, and physical characteristics of compounds in drug discovery; supporting the development and optimization of drug candidates. In this way, machine learning techniques can support the rapid identification and validation of stable compounds that meet predetermined structural requirements.
Structure-based drug design (SBDD)
3-dimensional in silico structures of drug targets can also be developed for interrogation. These can be used to analyze the physicochemical properties of target molecules, as well as aid in identifying key interactions. From this, computational tools can aid in understanding the shape and electronic interactions required of a molecule to bind and modulate the activity of the desired target.
Compounds are developed in a manner reminiscent of the “lock and key” model, aiming to complement the physicochemical properties of the target. By utilizing AI tools to predict the binding affinity and efficacy of drug candidates, based on structural information, computer models can inform drug candidate optimization strategies.
Computer models based on genetic libraries
SBDD and LBDD play critical roles in reducing the cost and time involved in the design and identification of drug molecules. In combination with high-throughput screening techniques, computer models are able to virtually analyze more than one million compounds in parallel. This allows developers to determine the structure of novel active small molecules that interact with desired targets.
Machine learning tools in combination with high-throughput screening and online multi-omic databases enable a deeper understanding of the chemical and biological activities of compounds. By utilizing databases such as PubChem and ChemSpider, information on drugs, targets and pathways can inform the discovery, development, optimization, and synthesis of drug candidates.
The PubChem open database provides a resource of over 100 million compounds, comprising the world's largest collection of freely accessible chemical information. Similarly, the ChemSpider library houses over 100 million structures, properties, and associated information. Providing a collection of unique chemical structures, as well as an aggregated view of known properties for a single chemical structure, these databases can inform computational models.
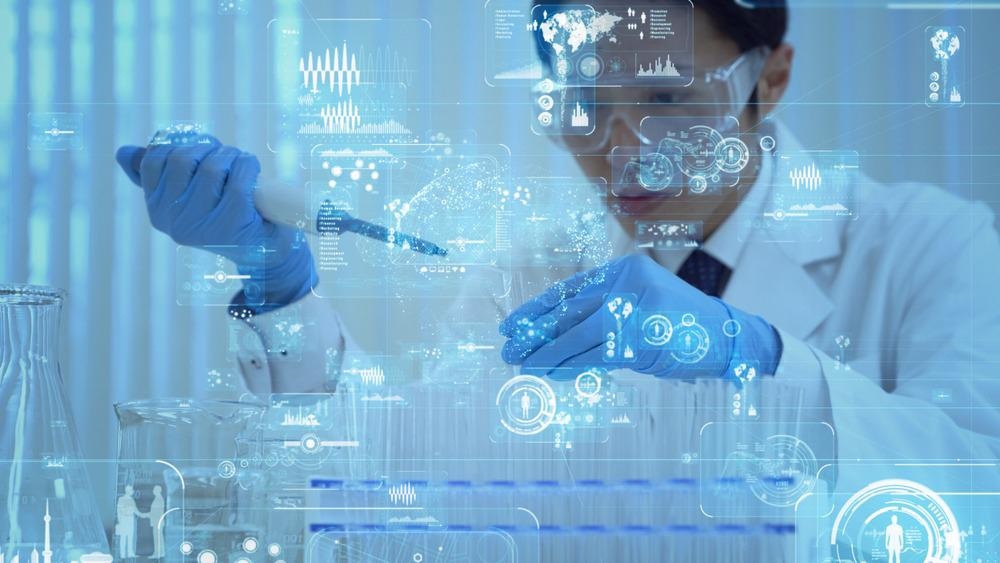
Image Credit: metamorworks/Shutterstock.com
Deep learning AI for statistic and predictive modeling
Deep learning networks can be applied to developing models from these databases. This approach allows for computers to be trained on datasets integrating structural, genetic, and pharmacological information; such that the efficacy of drug candidates can be virtually tested against modeled targets.
Considered a cutting-edge area of drug development, deep learning algorithms support the ability of computational models to learn from multidimensional data. QSAR analysis is a form of deep learning AI that aids in determining physicochemical descriptors of molecular activity. 3D-QSAR has enabled a deeper understanding of how physicochemical properties influence ligand-target interactions.
The accuracy of computational models in predicting the interaction of drug candidates with their target is largely determined by the quality of data input. While currently, these algorithmic approaches are not considered full proof or thoroughly capable, computer-aided drug discovery and development provide useful insights into the understanding of the binding affinity and molecular interaction between target protein and ligand.
Sources:
- Lin, X., Li, X., & Lin, X. (2020). A Review on Applications of Computational Methods in Drug Screening and Design. Molecules. https://doi.org/10.3390/molecules25061375
- Brogi, S., et al. (2020). Editorial: In silico Methods for Drug Design and Discovery. Front Chem. doi.org/10.3389/fchem.2020.00612
- Dara, S., et al. (2021). Machine Learning in Drug Discovery: A Review. Artificial intelligence review. doi.org/10.1007/s10462-021-10058-4
- Berg, J. M., et al. (2002). Biochemistry. New York: W.H. Freeman.
- Patel, L., et al. (2020). Machine Learning Methods in Drug Discovery. Molecules.. https://doi.org/10.3390/molecules25225277
- ChemSpider [Online]. Royal Society of Chemistry. Available at: http://www.chemspider.com/ (Accessed on 30 November 2021)
- PubChem [Online]. National Cenre for Biotechnology Information. Available at: https://pubchem.ncbi.nlm.nih.gov/ (Accessed on 30 November 2021)
Further Reading