Quantum computing is a new and exciting technology aimed at exploring the properties of quantum mechanics to unravel technical difficulties which classical computational methods have not solved.
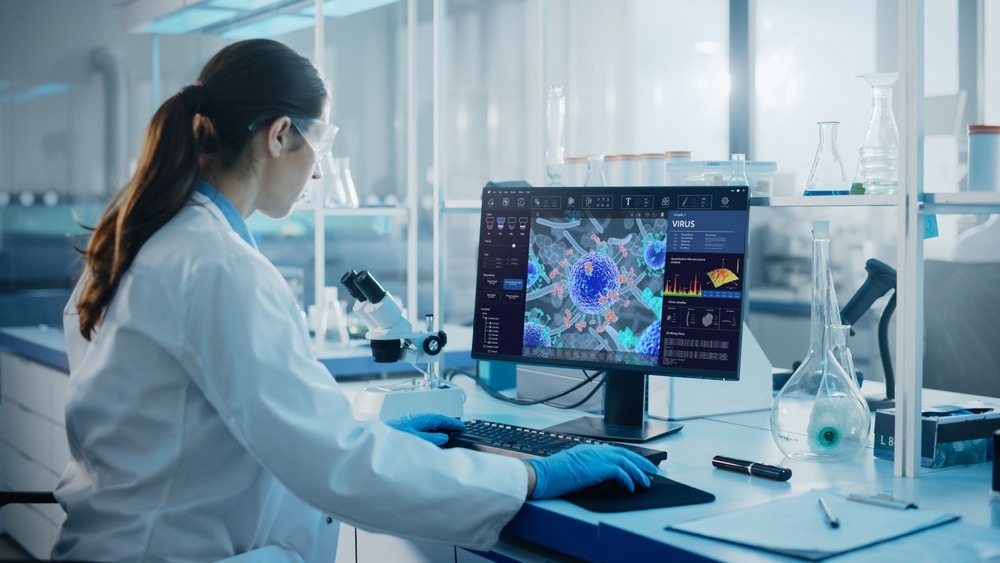
Image Credit: Gorodenkoff/Shutterstock.com
Drug discovery has greatly benefited from quantum computing by identifying novel drug targets and validating them at unprecedented speed. High-throughput in silico screening is the main quantum computing strategy for drug discovery. It enables the discovery of ligands that bind to specific molecular targets by an analytical procedure exhibiting high sensitivity and accuracy.
Quantum Computing in Drug Discovery
Drug discovery is defined as a research process involved in identifying and validating drugs that can be used to treat disease states and/or health conditions by meeting the highest safety and quality standards. Drug discovery normally goes through several sequential phases, including basic research, preclinical assays, clinical development studies, and regulatory approval. The first stage of drug discovery is often a complex, expensive, and time-consuming procedure that involves long computational runs and requires exhaustive computational analyses.
Quantum computing is a computer-driven strategy that exploits the underlying properties of matter and light to make analyses of data at a faster speed and higher accuracy than possible from any previously known methodology. These properties include superposition and interference, which supercomputers can exploit to perform high-scale operations capable of predicting outcomes that otherwise would be impossible to make using traditional computational methods.
In the drug discovery process, quantum computing guarantees to obtain accurate data projections considering many diverse biological parameters simultaneously. For example, quantum computing allows the analysis of a nearly infinite number of protein ligands that might eventually decrease the efficacy of a drug in vivo. This strategy has already shown to be very useful for protein modeling and the determination of drug candidates specific to the biological system of the study.
Quantum Algorithms vs. Quantum Circuits
A quantum algorithm is capable of running realistic models, being the quantum circuit computation model the most widely employed in the area of quantum computing. This benchmark is based on qubit state changes, where one qubit takes the value of two bits with quantum gates and on different qubit state measurements (2n values).
Conversely, quantum circuits refer to computing models that are analogous to traditional electrical circuits. In this case, data analysis is represented by linear sequences of quantum gates, qubits, and other types of computational measurements. Each quantum circuit is thus based on a computational routine on quantum data (e.g., qubits).
Machine Learning and Quantum Computing
The most widely used quantum Computing algorithms in drug discovery are known as machine learning. This strategy uses artificial intelligence systems to conduct sophisticated computational tasks and predict outcomes from a given data set.
Machine learning is based on statistical data and regression analyses, where classification and regression trees (CARTs) can be adapted for calculating computationally outcome-driven simulations. Some of the most commonly implemented quantum machine learning algorithms in drug discovery include, among others, naive Bayesian, support vector machines, deep neural networks, etc.
Schrödinger: Transforming drug discovery with high performance computing in the cloud
What are the Pros of Quantum Computing in Drug Development?
High Accuracy
Quantum Computing can significantly accelerate efforts in drug development by providing a highly plastic platform that can assess the performance of diverse drugs simultaneously, thereby enhancing costs and benefits.
Personalized Medicine
Quantum Computing uses machine learning algorithms that show a high predictive value for both large-scale and small-scale data, thereby enabling the detection of disease states with an extremely high percentage of chance (over 95 percent).
High Scalability
Quantum Computing platforms offer the ability to be adjusted in size and/or scale, which allows the discovery of drugs targeting major diseases (e.g., cancer, cardiovascular diseases, etc.) and also rare conditions by detecting point mutations associated with monogenic inherited disorders (e.g., spinal muscular atrophy, sickle-cell anemia, etc.).
The Future of Quantum Computing in Drug Development
The huge amount of already available information about potential drugs that were screened under different experimental conditions is such a complex process that it is almost impossible to assess by using classical computational approaches.
Quantum machine learning algorithms offer a chance to make this task affordable. These methods efficiently execute complex computational calculations on huge data sets with millions of samples, molecular descriptors, and differential parameters. Moreover, quantum computing can also quickly curate and update biological data sets as new screening info is available.
Nonetheless, it is also important to highlight that quantum computing raises some significant problems that must be addressed to be successfully used in medical settings. For example, from a purely chemical point of view, it is required to achieve a priori knowledge of how molecular predictors, i.e., the mathematical representations of molecules' properties developed by quantum computing algorithms, may hamper the ability of the model to make accurate predictions.
Sources:
- Batra, Kushal, et al. "Quantum machine learning algorithms for drug discovery applications." Journal of Chemical Information and Modeling 61.6 (2021): 2641-2647. DOI: https://doi.org/10.1021/acs.jcim.1c00166.
- Cao, Yudong, Jhonathan Romero, and Alán Aspuru-Guzik. "Potential of quantum computing for drug discovery." IBM Journal of Research and Development 62.6 (2018): 6-1. DOI: https://10.1147/JRD.2018.2888987.
- Pulkkis, Göran, and Kaj J. Grahn. "Current Quantum Information Science and Technology." Encyclopedia of Information Science and Technology, Third Edition. IGI Global, 2015. 4279-4292. DOI: https://10.4018/978-1-4666-5888-2.ch420.
- Zinner, Maximillian, et al. "Toward the institutionalization of quantum computing in pharmaceutical research." Drug Discovery Today (2021). DOI https://doi.org/10.1016/j.drudis.2021.10.006.
Further Reading