Classical or forward pharmacology, the traditional drug discovery method, is increasingly being replaced by the newer, structure-based drug design (SBDD) approach. In recent years, SBDD has established itself as a vital tool for pharmaceutical lead discovery that is more rapid and cost-effective than the traditional approach. Already, hundreds of new potential therapeutic targets have been identified in genomic, proteomic, and structural studies.
The future of drug discovery will likely rely on advancements in the area of SBDD to find new targets that could be developed into effective new therapeutics. As a result, the prognosis for various diseases may improve, as may diagnostic and screening techniques and treatment monitoring methods.
The success of SBDD poses one challenge, however, the significant number of targets it is uncovering is leading to a demand for approaches capable of processing such large data sets to extract meaningful information and make accurate predictions as to the therapeutic potential of a given target. In the last few years ‘big data’ has emerged in the field of drug discovery. Artificial intelligence (AI) and deep learning will continue to play an essential role in the analysis of data sets produced by SBDD.
Here, we give an overview of the process and future opportunities for SBDD.
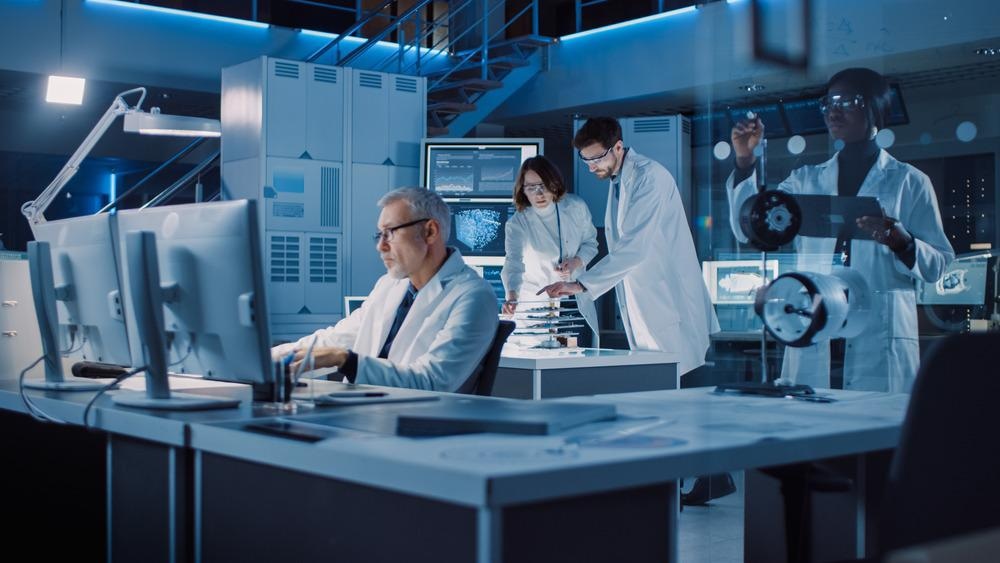
Image Credit: Gorodenkoff/Shutterstock.com
Overcoming the limitations of traditional drug design
The development of effective novel therapeutics relies on the interaction of a compound with a therapeutic target. Novel drugs are developed to interact with biological mechanisms, inducing certain outcomes by triggering biological actions or pathways. Via traditional methods of lead identification, outputs are produced by high-throughput screening (HTS). However, this process puts pressure on resources as it is time-consuming and expensive. The average drug takes roughly 14 years of development before it is approved by the FDA and will have cost around $800 million to make it there.
The traditional method of drug design is not only hindered by its time-consuming and costly nature, its high level of failure means that new drugs aren’t making it to market frequently enough. Only a small portion of potential drugs that begin the path to approval make it through, most are dropped at various stages of the drug development process, incurring further costs and leaving various patient populations with limited therapeutic options. There still remain many diseases that have no cure or no disease-modifying therapy available.
The SBDD method addresses these issues. It is relatively faster, cheaper, and has higher success rates than the traditional drug design method. The method is sometimes referred to as reverse pharmacology due to the reverse nature of its process. The first step involves target protein identification, once appropriate targets have been determined they are then screened against various libraries of small molecules, for those that complement the target in terms of its structure.
Recent advances in structural and molecular biology and biomolecular spectroscopic structure determination methods have helped to further the field of SBDD, resulting in the structural information of more than 100,000 proteins having been identified. Additionally, advances in AI and ‘big data’ have expanded our data storage and analytical processes, which has helped to improve our processes of target identification and screening.
The process of structure-based drug design
Computational resources are leveraged by the SBDD paradigm to enhance and accelerate the key screening processes, combinational chemistry, and the calculations of important properties such as absorption, distribution, excretion, metabolism, and toxicity. Due to its efficiencies in these areas, SBDD is considered to be the most powerful and efficient drug discovery paradigm currently in use.
SBDD is utilized throughout the drug discovery and development cycle, from identifying a target to designing a novel drug and entering it into clinical trials. To begin with, the target gene associated with the disease or symptom the therapeutic is intended to affect is identified, extracted, and purified. The 3D structure is then determined. Following this, computer algorithms are used to compare thousands of small molecules against the binding cavity of the identified target protein. Identified molecules are then ranked in terms of which are most likely to interact with the target site.
Molecules ranking highest are then synthesized and optimized and tested in-vitro in biochemical assays. Drugs are then developed based on the findings of these studies, and molecules that induce the desired therapeutic or pharmacological effect are further investigated. Usually, the structure of the ligand-protein complex is explored to develop multiple iterations of this binding to increase the chances of producing an effective novel therapeutic.
SBDD will likely continue to play an important role in the future of drug discovery. Traditional methods will probably further decrease in use as further advancements in SBDD and the fields that feed into it, such as biochemistry and AI, progress the paradigm.
Sources:
- Anderson, A., 2003. The Process of Structure-Based Drug Design. Chemistry & Biology, 10(9), pp.787-797. https://www.sciencedirect.com/science/article/pii/S1074552103001947
- Batool, M., Ahmad, B. and Choi, S., 2019. A Structure-Based Drug Discovery Paradigm. International Journal of Molecular Sciences, 20(11), p.2783. https://www.ncbi.nlm.nih.gov/pmc/articles/PMC6601033/
- Maia, E., Assis, L., de Oliveira, T., da Silva, A. and Taranto, A., 2020. Structure-Based Virtual Screening: From Classical to Artificial Intelligence. Frontiers in Chemistry, 8. https://www.frontiersin.org/articles/10.3389/fchem.2020.00343/full
Further Reading