An evolving RNA molecule can be cut and joined, or spliced, in various ways on the path from gene to protein before being translated into a protein. Using a technique called alternative splicing, multiple proteins can be encoded by a single gene.
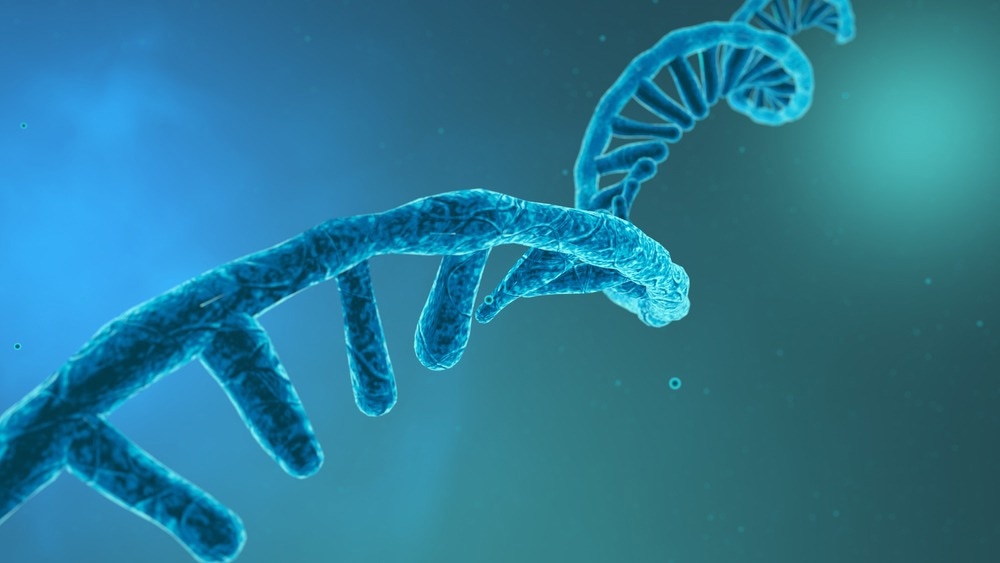
Image Credit: CROCOTHERY/Shutterstock.com
Many biological processes, such as the development of stem cells into tissue-specific cells, involve alternative splicing. However, alternative splicing can be dysregulated in the context of disease. To determine the underlying cause of a condition, it is crucial to examine the transcriptome or all RNA molecules that could result from a gene.
However, because RNA molecules typically have thousands of bases, it has historically been challenging to “read” them in their entirety. Instead, scientists have relied on a technique known as short-read RNA sequencing, which divides RNA molecules into much smaller units and then sequences them.
These units can range in size from 200 to 600 bases, depending on the platform and protocol. The complete sequences of the RNA molecules are then rebuilt using computer programs.
Short-read RNA sequencing can produce sequencing data that is extremely accurate, with a low per-base error rate of about 0.1%. (Meaning one base is incorrectly determined for every 1,000 bases sequenced). However, because the sequencing reads are so short, it is constrained in the amount of information they can offer.
In many ways, short-read RNA sequencing is similar to disassembling a large picture into numerous identically sized and shaped jigsaw puzzle pieces before attempting to reassemble the picture.
Recently, “long-read” platforms have become accessible, allowing for the end-to-end sequencing of RNA molecules longer than 10,000 bases. Although these platforms do not demand that RNA molecules be disassembled prior to sequencing, their per-base error rates are much higher, typically ranging from 5% to 20%.
Long-read RNA sequencing has been widely adopted, but this has been severely hampered by this well-known limitation. The high error rate has made it particularly challenging to assess the reliability of novel, previously unidentified RNA molecules found in a particular condition or disease.
Children’s Hospital of Philadelphia (CHOP) researchers have solved this issue by creating a new computational tool that can more precisely identify and quantify RNA molecules from these error-prone long-read RNA sequencing data.
It was described in the issue of Science Advances under the name ESPRESSO (Error Statistics PRomoted Evaluator of Splice Site Options).
Long-read RNA sequencing is a powerful technology that will allow us to uncover RNA variation in rare genetic diseases and other conditions, like cancer. We are probably at an inflection point in how we discover and analyze RNA molecules. The transition from short-read to long-read RNA sequencing represents an exciting technological transformation, and computational tools that reliably interpret long-read RNA sequencing data are urgently needed.”
Yi Xing, PhD, Study Senior Author and Director, Center for Computational and Genomic Medicine
Using just the error-prone long-read RNA sequencing data, ESPRESSO is capable of accurately identifying and quantifying various RNA molecules derived from the same gene, or RNA isoforms. To do this, a computational tool compares all long RNA sequencing reads from a gene to its corresponding genomic DNA.
It then uses the error patterns of individual long reads to convincingly identify splice junctions, which are locations where the nascent RNA molecule has been cut and joined, along with their corresponding full-length RNA isoforms.
The tool is able to identify highly reliable splice junctions and RNA isoforms, including those that have not yet been listed in existing databases, by looking for regions of perfect matches between long RNA sequencing reads and genomic DNA in addition to borrowing information across all long RNA sequencing reads of a gene.
ESPRESSO’s performance was assessed by the researchers using both data from actual biological samples and data from simulations. They discovered that ESPRESSO outperforms a number of currently available tools in terms of identifying and quantifying RNA isoforms.
The study of human transcriptome variation at the resolution of full-length RNA isoforms was aided by the researchers’ generation and analysis of over 1 billion long RNA sequencing reads from 30 different human tissue types and three different human cell lines.
Xing added, “ESPRESSO addresses a long-standing problem of long-read RNA sequencing and could usher in new opportunities of discovery. We envision that ESPRESSO will be a useful tool for researchers to explore the RNA repertoire of cells in various biomedical and clinical settings.”
Source:
Journal reference:
Gao, Y., et al. (2023). ESPRESSO: Robust discovery and quantification of transcript isoforms from error-prone long-read RNA-seq data. Science Advances. doi.org/10.1126/sciadv.abq5072